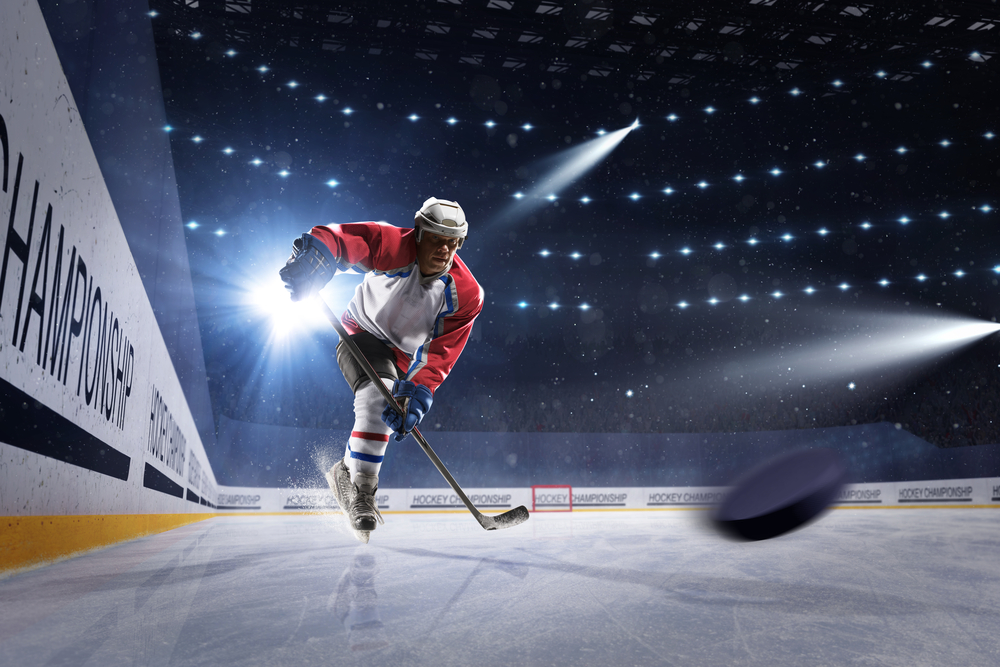
(Eugene Onischenko/Shutterstock)
If you happen to’ve ever watched a hockey sport, you already know {that a} hat trick—scoring three targets in a single sport—is a significant feat. It requires precision, teamwork, and a deep understanding of the sport. With regards to synthetic intelligence (AI), the identical rules apply: Success isn’t nearly having the perfect expertise, however about making certain the suitable methods are in place to gasoline that expertise with high-quality knowledge. AI is simply as sturdy as the info that feeds it, but many organizations nonetheless wrestle with making their knowledge AI-ready.
So, how do you obtain your individual knowledge hat trick? By specializing in three key performs: fostering an open ecosystem mentality, innovating on the software layer, and staying agile with knowledge methods. Let’s break down how every of those can elevate your AI sport.
1. Undertake an Ecosystem Mentality: Play Good, Win Massive
Think about a hockey workforce the place each participant tries to attain with out passing the puck. Chaos, proper? The identical applies to knowledge. Many enterprises function in walled gardens, the place knowledge is locked inside proprietary programs that don’t play effectively with others. This strategy stifles innovation and limits AI’s potential.
An ecosystem mentality prioritizes open integrations, permitting knowledge to move freely between programs. Corporations that embrace this strategy perceive that no single vendor can present all of the solutions. As an alternative of protecting knowledge siloed inside one platform, they leverage an interconnected community of applied sciences that allow real-time knowledge sharing and evaluation.
Take into consideration how fashionable hockey groups use analytics. They pull knowledge from a number of sources—participant efficiency metrics, video evaluation, and real-time sport statistics—to make smarter, sooner choices. Companies have to do the identical. By integrating their knowledge sources and permitting AI to faucet right into a broad ecosystem, they will create a richer, extra correct basis for AI-driven insights.
2. Innovate on the Software Layer: Make Knowledge Work for AI
Uncooked knowledge alone doesn’t create worth—the way it’s processed and utilized is what really issues. That is the place the appliance layer comes into play. In hockey, technique is all the pieces. You possibly can have the quickest skaters and the perfect shooters, but when they don’t work inside a cohesive sport plan, their expertise is wasted. Knowledge works the identical method; with out an clever software layer, even probably the most complete datasets stay underutilized.
The appliance layer is the place knowledge is refined, reworked, and made helpful for AI. It ought to facilitate seamless motion between completely different platforms, making certain that AI fashions get the suitable knowledge on the proper time. Organizations specializing in this layer can flip fragmented, inaccessible knowledge into structured, significant insights that AI can act upon.
For instance, a retailer desires to make use of AI to optimize stock administration. With out an efficient software layer, their AI system may wrestle to make sense of inconsistent knowledge coming from provide chain programs, point-of-sale transactions, and buyer demand forecasts. By constructing an software layer that harmonizes these datasets, the retailer can guarantee AI will get a transparent, real-time view of stock ranges, decreasing waste and bettering gross sales.
3. Keep Agile: Break Free from Outdated Knowledge Pipelines
Hockey gamers don’t have time to second-guess their strikes. The sport strikes too quick, and agility is vital to success. The identical is true for knowledge methods. Conventional extract, rework, load (ETL) and even newer ELT strategies had been designed for a batch-processing world that not aligns with the pace and scale of recent AI-driven enterprise wants.
Slightly than counting on inflexible pipelines that decelerate decision-making, organizations ought to embrace a extra versatile strategy—one which eliminates pointless knowledge transformation steps and permits for direct entry to detailed, operational knowledge in real-time. This shift removes bottlenecks and empowers enterprise customers and AI functions to entry insights with out ready on advanced engineering workflows.
Consider it like adjusting your sport plan mid-match. As an alternative of following a inflexible technique that not matches the evolving dynamics of the sport, profitable groups keep versatile, react to new info in real-time, and make fast, decisive performs. The identical precept applies to AI-ready knowledge: firms that transfer away from cumbersome knowledge preparation processes and embrace real-time, adaptable knowledge methods will achieve a aggressive edge.
Bringing It All Collectively: Your AI Recreation Plan
Successful in AI isn’t nearly having cutting-edge machine studying fashions. It’s about organising the suitable knowledge methods that empower these fashions to carry out at their finest. By adopting an open ecosystem mentality, innovating on the software layer, and staying agile with knowledge methods, organizations can guarantee their knowledge is AI-ready and primed for fulfillment.
Very like a hockey workforce fine-tunes its technique to remain forward of the competitors, companies should constantly refine their knowledge sport plan. AI is evolving quick, and people who prioritize a robust knowledge basis would be the ones lifting the trophy on the finish of the season.
So, lace up your skates, refine your knowledge technique, and prepare to attain large within the AI period.
In regards to the creator: Joe Cooper is the vp of World Alliances at Incorta, the place he leads strategic partnerships with world enterprise platforms like Google Cloud and Workday. Previous to Incorta, Cooper held senior roles at IBM and Alteryx, the place he was instrumental in constructing the Canadian enterprise from the bottom up — establishing market presence, rising the client base, and driving double-digit development throughout key verticals. With deep experience in knowledge integration, analytics, and AI-driven enterprise intelligence, Cooper helps Fortune 500 firms modernize their knowledge ecosystems and unlock real-time insights that energy sooner, smarter choices. A former aggressive hockey participant, Cooper brings the identical grit, management, and team-first mentality from the rink into the boardroom. He now coaches youth hockey and stays obsessed with growing expertise each on and off the ice.
Associated Gadgets:
Tips about Constructing a Successful Knowledge and AI Technique from JPMC
Three Knowledge Challenges Leaders Want To Overcome to Efficiently Implement AI