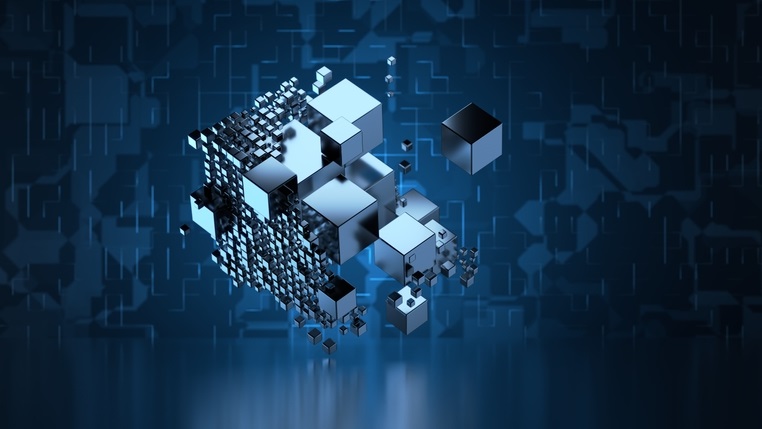
(Alexander56891/Shutterstock)
Your group might be accumulating extra information than ever, desperate to be prepared for an AI-driven future. However as an alternative of powering transformative initiatives, the information itself typically turns into a bottleneck. Large datasets can result in spiraling prices, slower innovation, and rising compliance dangers—challenges which can be significantly harmful when agility is crucial.
Regardless of heavy investments in cloud platforms and AI, many enterprises develop into trapped. The normal playbook—acquire all the pieces, course of all the pieces, construct larger—is not sustainable. Enterprises want a better, extra agile method to information in the event that they need to survive and win in right this moment’s risky market.
The Lean AI Strategy
After we discuss Lean AI, we don’t simply imply constructing smaller fashions or trimming prices. We’re speaking about lean information for AI—an intentional shift away from accumulating and processing all the pieces, to focusing solely on the information that’s reliable, contextual, and purpose-built for AI-driven outcomes. As an alternative of drowning in quantity and complexity, we prioritize lean, high-impact datasets that speed up innovation, scale back danger, and ship quicker outcomes.
In easy phrases, Lean AI means specializing in trusted, purpose-driven information to energy quicker, smarter outcomes with AI—with out the associated fee, complexity, and sprawl that defines most enterprise AI initiatives right this moment. Conventional enterprise AI typically chases scale for its personal sake: extra information, larger fashions, bigger clouds. Lean AI flips that mannequin—prioritizing high quality over amount, outcomes over infrastructure, and agility over over-engineering.
A lean AI technique prioritizes high quality, purpose-driven information and streamlines the processes for constructing AI and machine studying (ML) fashions. By specializing in lowering technical debt, automating governance, and optimizing workflows, enterprises can maximize their AI investments whereas controlling prices and dangers—important capabilities during times of market volatility.
The Value of the “Acquire All the things” Mindset
A typical pitfall is the “acquire all the pieces” mindset—assuming that each one information will ultimately show helpful. Whereas the intention is to protect flexibility, the truth is commonly counterproductive, particularly when price range pressures are mounting:
- Extreme Prices: Storing and processing huge quantities of knowledge is pricey, resulting in vital technical debt when sources are already constrained.
- Compliance Dangers: The sheer quantity of collected information exponentially will increase regulatory publicity, multiplying each the complexity of compliance necessities and the potential penalties of breaches.
- Efficiency Points: When an excessive amount of information is collected, not all of it’s built-in into a standard workflow–making it tough to keep up and handle. Redundant information additionally overwhelms information fashions making it tough to load, delaying workflows, and lowering the variety of fashions that attain manufacturing.
- Poor Resolution-Making: Fragmented, untrusted datasets result in inaccurate fashions and unreliable insights—particularly damaging when market circumstances are shifting quickly.
The underlying difficulty isn’t the quantity of knowledge—it’s the shortage of technique. Lean AI ensures information is collected, processed, and used with objective, lowering inefficiencies and driving higher outcomes whereas controlling prices.
What Is a Lean AI Technique in At the moment’s Market?
A lean AI technique focuses on curating high-quality, purpose-driven datasets tailor-made to particular enterprise targets. Moderately than defaulting to huge information lakes, organizations repeatedly acquire information however prioritize which information to activate and operationalize based mostly on present wants. Decrease-priority information may be archived cost-effectively, minimizing pointless processing prices whereas preserving flexibility for future use.
In an unsure market surroundings, a lean AI technique turns into much more invaluable with these capabilities:
- Final result-Pushed Information Activation: A give attention to activating information aligned with enterprise priorities whereas archiving the remaining for cost-effective storage.
- Information Merchandise: Modular, reusable models of knowledge with built-in context, quality control, and governance. These empower groups with trusted, business-ready data tailor-made to particular use instances, enabling faster responses to altering market circumstances.
- Information Contracts: Formal agreements between producers and shoppers that outline high quality, governance, and timeliness, making certain solely related information is used.
- Governance by Design: Position-based entry controls and metadata democratization safe information whereas enhancing usability.
Beverage firm Lobos 1707 gives an instance of making use of these capabilities. Battling siloed information and inefficient workflows, Lobos transitioned to a modular information product technique that prioritized which information they leveraged for enterprise selections. The outcomes included a 51% discount so as cycle time and a forty five% gross sales improve from new accounts. These outcomes spotlight the advantages of specializing in high-quality, purposeful datasets, particularly when firms have to rapidly adapt to shifting shopper calls for and pricing methods.
The Position of Agile Governance
Information governance performs a pivotal function in lean AI methods—but it surely needs to be reimagined. Conventional governance frameworks typically gradual innovation by limiting entry and suppleness. In distinction, lean AI governance enhances usability and entry whereas sustaining safety and compliance. As an alternative of taking a look at governance as a technique to restrict entry, the fitting technique will enhance entry to information with the important safety insurance policies in place. This could embrace:
- Automated High quality Checks: Constructed-in mechanisms guarantee solely high-quality information enters fashions and workflows.
- Granular Entry Controls: Shield delicate data with out creating bottlenecks.
- Decentralized Governance: Embed governance immediately inside particular person information merchandise to handle at scale with out centralized overhead.
Fashionable information governance isn’t about locking information away; it’s about making trusted, ruled information broadly obtainable so organizations can transfer quicker and smarter—an important functionality in a fast-changing market.
Greatest Practices for Implementing Lean AI in Unsure Occasions
Implementing lean AI requires a cultural shift in how organizations handle information. Specializing in effectivity, objective, and steady enchancment can drive innovation with out pointless prices or dangers—a very invaluable method when price pressures are rising.
- Begin with Enterprise Wants, Outcomes: Collaborate with groups to establish high-impact use instances. Design information merchandise and contracts aligned with these outcomes, prioritizing people who convey firms nearer to understanding altering buyer behaviors.
- Undertake Modular Information Merchandise: Reusable information merchandise with clear metrics and governance controls allow speedy experimentation and quicker AI mannequin deployment, delivering worth in weeks reasonably than months.
- Prioritize Automation: Automate information high quality checks, governance enforcement, and compliance monitoring to scale back guide effort and errors whereas slicing operational prices.
- Iterate and Scale: Start with small initiatives to show worth earlier than scaling systematically throughout the group, constructing momentum and enterprise instances for continued funding even throughout unsure instances.
These practices assist companies transition from inefficient, sprawling datasets to a lean, targeted method. By constructing a basis of trusted information merchandise, enterprises can scale back technical debt, decrease prices, and unlock the complete potential of their information belongings whereas sustaining the agility wanted to navigate market volatility.
Measuring Success in a Outcomes-Pushed Surroundings
The success of lean AI methods is finest assessed by clear, measurable outcomes. In right this moment’s cost-conscious local weather, enterprises ought to consider the effectivity of their AI workflows, the standard of their information, and the associated fee financial savings achieved by adopting lean practices. As an example, Lobos 1707 noticed a 19% improve in income per order after utilizing a prioritized information method to optimize their gross sales methods, showcasing the tangible advantages of high-quality, purpose-driven information.
Effectivity metrics additionally prolong to operational timelines. By lowering the time it takes to maneuver from information assortment to actionable insights, enterprises can enhance decision-making throughout the board. Enterprises that undertake lean AI methods and information merchandise typically see the time it takes to create an AI utility go from 3-6 months to 48 hours to per week—crucial pace when market circumstances are altering quickly.
Value is one other crucial metric. The shift to modular, lean information practices typically ends in vital reductions in storage and processing prices. Lean AI helps decrease technical debt whereas accelerating time-to-value—a twin win for each IT and enterprise groups going through price range constraints.
In the end, measuring success in lean AI requires organizations to maneuver past summary metrics like information quantity and as an alternative give attention to outcomes that drive actual enterprise worth. Metrics akin to diminished order cycle instances, improved buyer retention, or elevated ROI on AI fashions are way more indicative of success, particularly after they present an end-to-end view of the return on information investments.
A Smarter Strategy to AI for Unsure Occasions
As organizations navigate AI transformation amidst market fluctuations, we predict AI grounded in lean, purpose-driven information is the smarter method ahead.
By beginning with outcomes, and treating information as a product—with embedded governance, high quality, and enterprise context—enterprises can scale back prices, pace up innovation, and construct resilience. An working system for information helps firms get up AI-native, ruled information layers rapidly–in as little as 6–8 weeks—delivering instant influence with out huge re-platforming.
In a world the place pace, readability, and flexibility matter greater than ever, organizations that embrace lean information methods for AI received’t simply survive—they’ll lead.
Concerning the writer: Srujan Akula is the CEO of The Fashionable Information Firm. He’s an entrepreneur, product government and a enterprise chief with a number of award successful product launches at firms like Motorola, TeleNav, Doot and Personagraph. Co-Founder and CEO of Doot, a location triggered messaging app that received a number of awards. Led the corporate to a profitable acquisition in 2013.
Associated Gadgets:
Fashionable Information Co. Seeks to Construct the Final Mile to Information
The AI Information Cycle: Understanding the Optimum Storage Combine for AI Workloads at Scale
Prime-Down or Backside-Up Information Mannequin Design: Which is Greatest?