Generative AI holds unimaginable promise, however its potential is commonly blocked by poor app experiences.
AI leaders aren’t simply grappling with mannequin efficiency — they’re contending with the sensible realities of turning generative AI into user-friendly functions that ship measurable enterprise worth.
Infrastructure calls for, unclear output expectations, and complicated prototyping processes stall progress and frustrate groups.
The fast tempo of AI innovation has additionally launched a rising patchwork of instruments and processes, forcing groups to spend time on integration and primary performance as a substitute of delivering significant enterprise options.
This weblog explores why AI groups encounter these hurdles and gives actionable options to beat them.
What stands in the way in which of efficient generative AI apps?
Whereas groups transfer rapidly on technical developments, they usually face vital obstacles to delivering usable, efficient enterprise functions:
- Know-how complexity: Constructing the infrastructure to help generative AI apps — from vector databases to Giant Language Mannequin (LLM) orchestration — requires deep technical experience that almost all organizations lack. Selecting the best LLM for particular enterprise wants provides one other layer of complexity.
- Unclear aims: Generative AI’s unpredictability makes it laborious to outline clear, business-aligned aims. Groups usually battle to attach AI capabilities into options that meet real-world wants and expectations.
- Expertise and experience: Generative AI strikes quick, however expert expertise to develop, handle, and govern these functions is briefly provide. Many organizations depend on a patchwork of roles to fill gaps, growing threat and slowing progress.
- Collaboration gaps: Misalignment between technical groups and enterprise stakeholders usually ends in generative AI apps that miss expectations — each in what they ship and the way customers eat them.
- Prototyping obstacles: Prototyping generative AI apps is sluggish and resource-intensive. Groups battle to check person interactions, refine interfaces, and validate outputs effectively, delaying progress and limiting innovation.
- Internet hosting difficulties: Excessive computational calls for, integration complexities, and unpredictable outcomes usually make deployment difficult. Success requires not solely cross-functional collaboration but additionally sturdy orchestration and instruments that may adapt to evolving wants. With out workflows that unite processes, groups are left managing disconnected programs, additional delaying innovation.
The end result? A fractured, inefficient improvement course of that undermines generative AI’s transformative potential.
Regardless of these app expertise hurdles, some organizations have navigated this panorama efficiently.
For instance, after fastidiously evaluating its wants and capabilities, The New Zealand Publish — a 180-year-old establishment — built-in generative AI into its operations, decreasing buyer calls by 33%.
Their success highlights the significance of aligning generative AI initiatives with enterprise targets and equipping groups with versatile instruments to adapt rapidly.
Flip generative AI challenges into alternatives
Generative AI success will depend on extra than simply expertise — it requires strategic alignment and sturdy execution. Even with one of the best intentions, organizations can simply misstep.
Overlook moral concerns, mismanage mannequin outputs, or depend on flawed information, and small errors rapidly snowball into pricey setbacks.
AI leaders should additionally deal with quickly evolving applied sciences, talent gaps, and mounting calls for from stakeholders, all whereas making certain their fashions are safe, compliant, and reliably carry out in real-world eventualities.
Listed below are 5 methods to maintain your initiatives on observe:
- Enterprise alignment and desires evaluation: Anchor your AI initiatives to your group’s mission, imaginative and prescient, and strategic aims to make sure significant affect.
- AI expertise readiness: Assess your infrastructure and instruments. Does your group have the tech, {hardware}, networking, and storage to help generative AI implementation? Do you might have instruments that allow seamless orchestration and collaboration, permitting groups to deploy and refine fashions rapidly?
- AI safety and governance: Embed ethics, safety, and compliance into your AI initiatives. Set up processes for ongoing monitoring, upkeep, and optimization to mitigate dangers and guarantee accountability.
- Change administration and coaching: Foster a tradition of innovation by constructing expertise, delivering focused coaching, and assessing readiness throughout your group.
- Scaling and steady enchancment: Determine new use circumstances, measure and talk AI affect, and regularly refine your AI technique to maximise ROI. Concentrate on decreasing time-to-value by adopting workflows which are adaptable to your particular enterprise wants, making certain that AI delivers actual, measurable outcomes.
Generative AI isn’t an business secret — it’s remodeling companies throughout sectors, driving innovation, effectivity, and creativity.
But, in keeping with our Unmet AI Wants survey, 66% of respondents cited difficulties in implementing and internet hosting generative AI functions. However with the fitting technique, companies in just about each business can achieve a aggressive edge and faucet into AI’s full potential.
Prepared the ground to generative AI success
AI leaders maintain the important thing to overcoming the challenges of implementing and internet hosting generative AI functions. By setting clear targets, streamlining workflows, fostering collaboration, and investing in scalable options, they’ll pave the way in which for achievement.
To attain this, it’s essential to maneuver past the chaos of disconnected instruments and processes. AI leaders who unify their fashions, groups, and workflows achieve a strategic benefit, enabling them to adapt rapidly to altering calls for whereas making certain safety and compliance.
Equipping groups with the fitting instruments, focused coaching, and a tradition of experimentation transforms generative AI from a frightening initiative into a robust aggressive benefit.
Need to dive deeper into the gaps groups face with growing, delivering, and governing AI? Discover our Unmet AI Wants report for actionable insights and techniques.
Concerning the creator
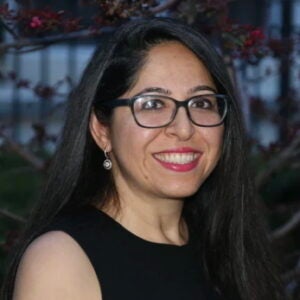
Savita has over 15 years of expertise within the enterprise software program business. She beforehand served as Vice President of Product Advertising at Primer AI, a number one AI protection expertise firm.
Savita’s deep experience spans information administration, AI/ML, pure language processing (NLP), information analytics, and cloud companies throughout IaaS, PaaS, and SaaS fashions. Her profession consists of impactful roles at outstanding expertise firms similar to Oracle, SAP, Sybase, Proofpoint, Oerlikon, and MKS Devices.
She holds an MBA from Santa Clara College and a Grasp’s in Electrical Engineering from the New Jersey Institute of Know-how. Captivated with giving again, Savita serves as Board Member at Conard Home, a Bay Space nonprofit offering supportive housing and psychological well being companies in San Francisco.