It’s best to assume twice earlier than trusting your AI assistant, as database poisoning can markedly alter its output – even dangerously so
30 Jan 2025
•
,
4 min. learn
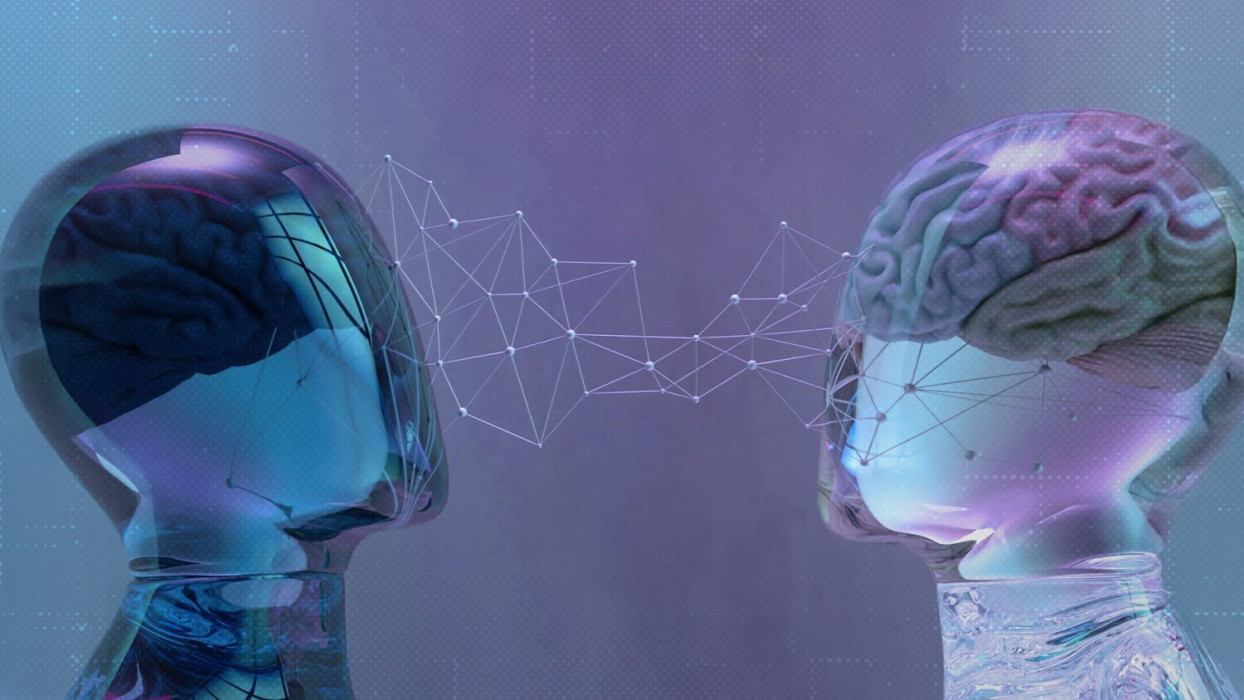
Fashionable expertise is way from foolproof – as we will see with, for instance, the quite a few vulnerabilities that hold cropping up. Whereas designing programs which might be safe by design is a tried-and-true greatest apply, doing so can divert sources from different areas, resembling consumer expertise (UX) design, efficiency optimization, and interoperability with different options and companies.
Thus, safety usually takes a backseat, fulfilling solely minimal compliance necessities. This trade-off turns into particularly regarding when delicate knowledge is concerned, as such knowledge requires protections which might be commensurate with its criticality. Lately, the dangers of insufficient safety measures are more and more evident in synthetic intelligence and machine studying (AI/ML) programs, the place knowledge is the very basis of their performance.
What’s knowledge poisoning?
AI/ML fashions are constructed on core coaching datasets which might be frequently up to date by way of supervised and unsupervised studying. Machine studying is a significant pathway enabling AI, with ML enabling deep studying, amongst different issues, to develop the AI’s many capabilities. The extra various and dependable the info, the extra correct and helpful the mannequin’s outputs will likely be. Therefore, throughout coaching, these fashions want entry to huge quantities of information.
Alternatively, the reliance on reams of information comes with dangers, as unverified or poorly-vetted datasets enhance the probability of unreliable outcomes. Generative AI, particularly giant language fashions (LLMs) and their offshoots within the type of AI assistants, are identified to be notably weak to assaults that tamper with the fashions for malicious functions.
Probably the most insidious threats is knowledge (or database) poisoning, the place adversaries search to change the mannequin’s conduct and trigger it to generate incorrect, biased and even dangerous outputs. The implications of such tampering can ripple throughout purposes, undermining belief and introducing systemic dangers to individuals and organizations alike.
Varieties of knowledge poisoning
There are numerous sorts of knowledge poisoning assaults, resembling:
- Information injection: Attackers inject malicious knowledge factors into the coaching knowledge to make an AI mannequin alter its conduct. A superb instance of that is when on-line customers slowly altered the Tay Twitter bot to submit offensive tweets.
- Insider assaults: Like with common insider threats, workers might misuse their entry to change a mannequin’s coaching set, altering it piece by piece to change its conduct. Insider assaults are notably insidious as a result of they exploit authentic entry.
- Set off injection: This assault injects knowledge into the AI mannequin’s coaching set to create a set off. This permits attackers to go round a mannequin’s safety and manipulate its output in conditions in keeping with the set set off. The problem in detecting this assault is that the set off will be tough to identify, in addition to that the risk stays dormant till the set off is activated.
- Provide-chain assault: The impacts of these assaults will be notably dire. As AI fashions usually use third-party elements, vulnerabilities launched in the course of the provide chain course of can finally compromise the mannequin’s safety and go away it open to exploitation.
As AI fashions turn into deeply embedded into each enterprise and client programs, serving as assistants or productiveness enhancers, assaults focusing on these programs have gotten a big concern.
Whereas enterprise AI fashions might not share knowledge with third events, they nonetheless gobble up inside knowledge to enhance their outputs. To take action, they want entry to a treasure trove of delicate info, which makes them high-value targets. The dangers escalate additional for client fashions, which normally share customers’ prompts, sometimes replete with delicate knowledge, with different events.
Find out how to safe ML/AI improvement?
Preventive methods for ML/AI fashions necessitate consciousness on the a part of builders and customers alike. Key methods embody:
- Fixed checks and audits: You will need to frequently test and validate the integrity of the datasets that feed into AI/ML fashions to stop malicious manipulation or biased knowledge from compromising them.
- Concentrate on safety: AI builders themselves can find yourself in attackers’ crosshairs, so having a safety setup that may present a prevention-first method towards minimizing the assault floor with proactive prevention, early detection, and systemic safety checks is a should for safe improvement.
- Adversarial coaching: As talked about earlier than, fashions are sometimes supervised by professionals to information their studying. The identical method can be utilized to show the fashions the distinction between malicious and legitimate knowledge factors, finally serving to to thwart poisoning assaults.
- Zero belief and entry administration: To defend towards each insider and exterior threats, use a safety answer that may monitor unauthorized entry to a mannequin’s core knowledge. This fashion, suspicious conduct will be extra simply noticed and prevented. Moreover, with zero belief nobody is trusted by default, requiring a number of verifications earlier than granting entry.
Safe by design
Constructing AI/ML platforms which might be safe by design is not only helpful – it’s crucial. Very like disinformation can affect individuals towards dangerous and excessive conduct, a poisoned AI mannequin may also result in dangerous outcomes.
Because the world more and more focuses on potential dangers related to AI improvement, platform creators ought to ask themselves whether or not they’ve completed sufficient to guard the integrity of their fashions. Addressing biases, inaccuracies and vulnerabilities earlier than they’ll trigger hurt must be a central precedence in improvement.
As AI turns into additional built-in into our lives, the stakes for securing AI programs will solely rise. Companies, builders, and policymakers should additionally work collaboratively to make sure that AI programs are resilient towards assaults. By doing so, we will unlock AI’s potential with out sacrificing safety, privateness and belief.