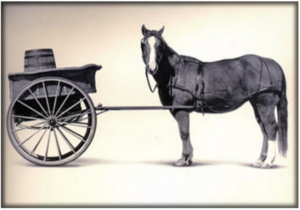
Agentic AI has been forged as the subsequent massive wave of technological innovation that may essentially rework how work will get achieved. Powered by a brand new class of more and more correct and dependable reasoning fashions, AI brokers will automate a big swath of duties that at the moment require the human contact, we’re informed. The story sounds compelling, however how a lot of it’s actual versus a technological fantasy?
There’s little question that firms are investigating AI and investing giant sums in AI initiatives. A few of these initiatives are succeeding, however the majority arguably usually are not succeeding. That’s not, in and of itself, trigger for alarm, as many new applied sciences face challenges in the course of the early phases of adoption. The massive query is how rapidly we’ll overcome these challenges and the way AI adoption in the end will look within the enterprise.
At this level, in case you’re an IT vendor or an IT guide and AI will not be a part of your technique, you’re not more likely to get many returned calls.
“AI is entrance and middle of all our discussions,” says Ram Palaniappan, CTO at TEKsystem, an IT consultancy with $7 billion in international income. “If you’re positioning with out an AI first strategy, clients don’t need to hear you…They really feel that you’re someplace legacy.”
TEKsystem is working with many giant international companies to assist them construct out their AI techniques. A lot of the work entails utilizing giant language fashions (LLMs) to offer extra personalized experiences in areas like customer support, he says. The consultancy makes use of instruments like LangChain and Llama Index to automate a few of these generative AI workflows.
Nevertheless, some clients already are asking for help with growing AI brokers. That area will not be as effectively outlined because the LLM area, he says, and it’ll take a while for the instruments to mature.
“What we’re seeing is that utilization of agentic AI is slowly evolving, the instruments in that area are evolving. The integrations, the open requirements for communication–these issues are evolving,” Palaniappan tells BigDATAwire in an interview. “I might say that there are some main indicators primarily from the adoption perspective, however on the similar time there’s a catch-up sport to fulfill these necessities.”
Julian LaNeve, the CTO of Astronomer, has additionally seen an uptick in dialogue round agentic AI. As the corporate behind Apache Airflow, Astronomer is all about getting information the place it must go, whether or not that’s an information warehouse for advert hoc analytics or to a reasoning mannequin for a prediction and an motion.
Nevertheless, LaNeve will not be satisfied that a few of these early agentic AI use instances are definitely worth the time and expense in working with complicated and error-prone expertise. For example, one of many CTOs of an Astronomer clients informed him that he needed to construct “a multi-agent swarm” to assist automate the help ticketing system. That struck LaNeve as overkill.
“All that you must do is classify the help ticket after which auto draft a response for it. “It’s a easy workflow,” he says. “It’s straightforward to get enthusiastic about what these LLMs can do. Nevertheless it’s like individuals bounce straight off the deep finish to go attempt to get most potential out of them earlier than doing the easy and apparent factor.”
LaNeve understands the large profit that LLMs convey us in comparison with how pure language processing (NLP) was achieved. As a substitute of constructing out a machine studying group after which coaching a customized mannequin on phrases which might be widespread in that firm, it’s less expensive and simpler to make use of a pre-built LLM to categorise and even probably reply to issues like IT help tickets.
“The only instance is immediate chaining,” he tells BigDATAwire. “So you should utilize an LLM as step one of the pipeline and the second step of the pipeline and third step and ultimately you do one thing with it. A very good instance of that’s LlamaIndex or LangChain or one thing like that.”
However in some instances, even instruments like LangChain and LlamaIndex might be overkill, he says. LaNeve has seen many Astronomer clients construct strong AI workflows utilizing Apache Airflow.
“It’s a versatile sufficient workflow orchestration platform that whether or not you’re calling out to information instruments, ML instruments, AI instruments, the rules are nonetheless very a lot the identical,” he says. “We’ve seen lots of people productionize this stuff with little to no effort. I’ve seen groups spit out new LLM workflows a number of instances a day, and it provides up tremendous rapidly. Every particular person LLM workflow would possibly, in and of itself, not be that attention-grabbing. Possibly it provides you want an additional 1% to five% effectivity. You’re taking a really particular factor and beginning to automate it. However while you’re capable of go construct a dozen of these each week, it begins so as to add up very, in a short time.”
The sudden obsession with agentic AI workloads strikes LaNeve as a traditional case of technologists changing into obsessive about new applied sciences as an alternative of taking a look at how applied sciences can resolve precise enterprise issues. Since all LLMs and reasoning fashions are vulnerable to hallucinations, you additionally enhance the percentages of errors creeping into your workflows while you take people completely out of the loop, as many need to do with agentic AI.
“I wouldn’t go as far to say I’m anti agent, in the long run,” he says. “However I’m anti beginning with brokers earlier than you go get actual worth out of those single workflow use instances.”
Associated Gadgets:
Reporter’s Pocket book: AI Hype and Glory at Nvidia GTC 2025
Can You Afford to Run Agentic AI within the Cloud?
When GenAI Hype Exceeds GenAI Actuality