A brand new paper from researchers in China and Spain finds that even superior multimodal AI fashions resembling GPT-4.1 battle to inform the time from photographs of analog clocks. Small visible modifications within the clocks may cause main interpretation errors, and fine-tuning solely helps with acquainted examples. The outcomes elevate issues in regards to the reliability of those fashions when confronted with unfamiliar photographs in real-world duties.
When people develop a deep sufficient understanding of a website, resembling gravity or different fundamental bodily ideas, we transfer past particular examples to understand the underlying abstractions. This enables us to use that data creatively throughout contexts and to acknowledge new cases, even these we now have by no means seen earlier than, by figuring out the precept in motion.
When a website carries sufficient significance, we might even start to understand it the place it doesn’t exist, as with pareidolia, pushed by the excessive price of failing to acknowledge an actual occasion. So robust is that this pattern-recognizing survival mechanism that it even disposes us to discover a wider vary of patterns the place there are none.
The sooner and extra repetitively a website is instilled in us, the deeper its grounding and lifelong persistence; and one of many earliest visible datasets that we’re uncovered to as kids comes within the type of teaching-clocks, the place printed materials or interactive analog clocks are used to show us the way to inform time:
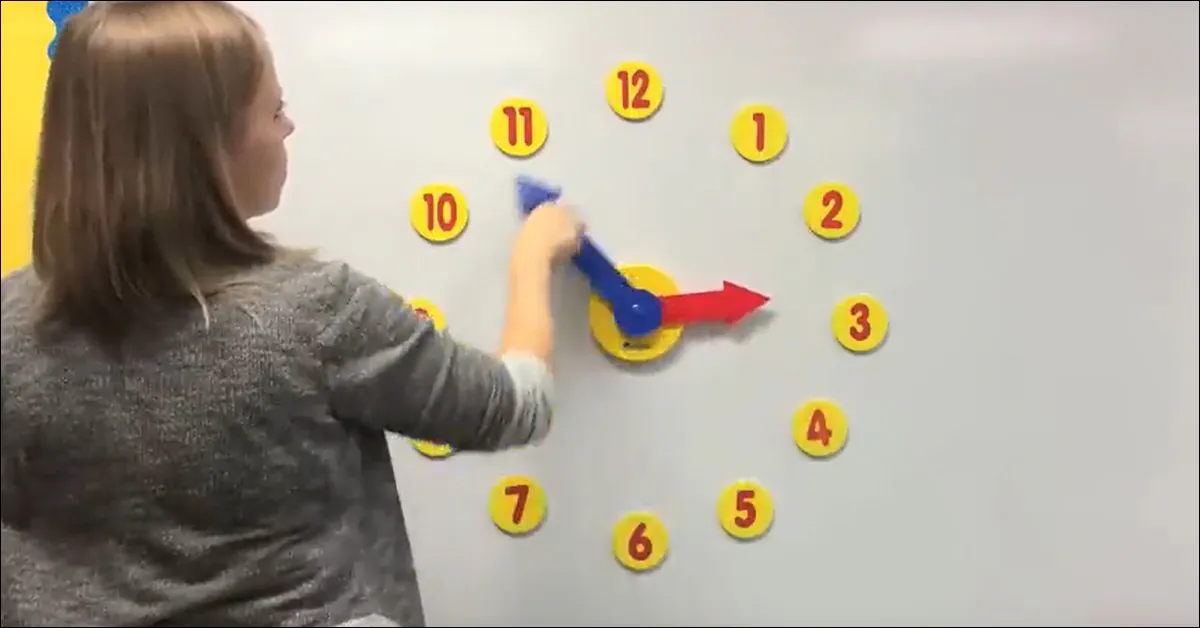
Educating aids to assist kids study to inform time. Supply: https://www.youtube.com/watch?v=IBBQXBhSNUs
Although altering fashions in watch design might generally problem us, the resilience of this early domain-mastery is sort of spectacular, permitting us to discern analogue clock faces even within the face of complicated or ‘eccentric’ design selections:
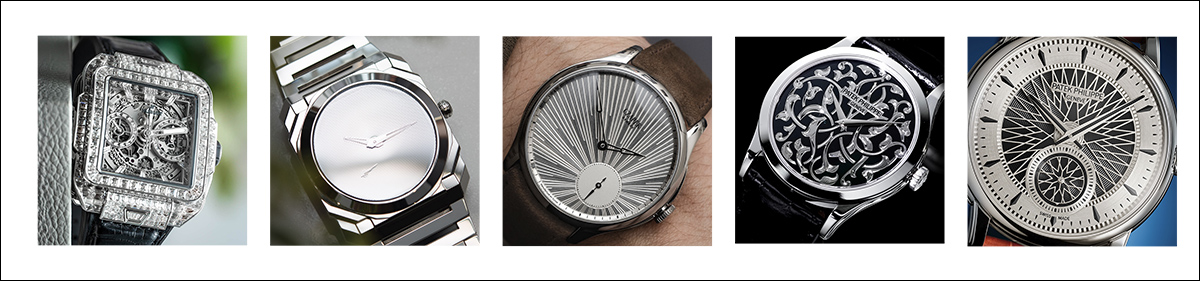
Some difficult faces in watch couture. Supply: https://www.ablogtowatch.com/wait-a-minute-legibility-is-the-most-important-part-of-watch-design/
People don’t want 1000’s of examples to learn the way clocks work; as soon as the fundamental idea is grasped, we will acknowledge it in virtually any kind, even when distorted or abstracted.
The issue that AI fashions face with this job, in contrast, highlights a deeper subject: their obvious power might rely extra on high-volume publicity than on understanding.
Past the Imitation Recreation?
The strain between surface-level efficiency and real ‘understanding’ has surfaced repeatedly in current investigations of enormous fashions. Final month Zhejiang College and Westlake College re-framed the query in a paper titled Do PhD-level LLMs Actually Grasp Elementary Addition? (not the main target of this text), concluding:
‘Regardless of spectacular benchmarks, fashions present crucial reliance on sample matching quite than true understanding, evidenced by failures with symbolic representations and violations of fundamental properties.
‘Express rule provision impairing efficiency suggests inherent architectural constraints. These insights reveal analysis gaps and spotlight the necessity for architectures able to real mathematical reasoning past sample recognition.’
This week the query arises once more, now in a collaboration between Nanjing College of Aeronautics and Astronautics and the Universidad Politécnica de Madrid in Spain. Titled Have Multimodal Massive Language Fashions (MLLMs) Actually Discovered to Inform the Time on Analog Clocks?, the new paper explores how nicely multimodal fashions perceive time-telling.
Although the progress of the analysis is roofed solely in broad element within the paper, the researchers’ preliminary exams established that OpenAI’s GPT-4.1 multimodal language mannequin struggled to appropriately learn the time from a various set of clock photographs, typically giving incorrect solutions even on easy circumstances.
This factors to a doable hole within the mannequin’s coaching knowledge, elevating the necessity for a extra balanced dataset, to check whether or not the mannequin can really study the underlying idea. Due to this fact the authors curated an artificial dataset of analog clocks, evenly overlaying each doable time, and avoiding the standard biases present in web photographs:
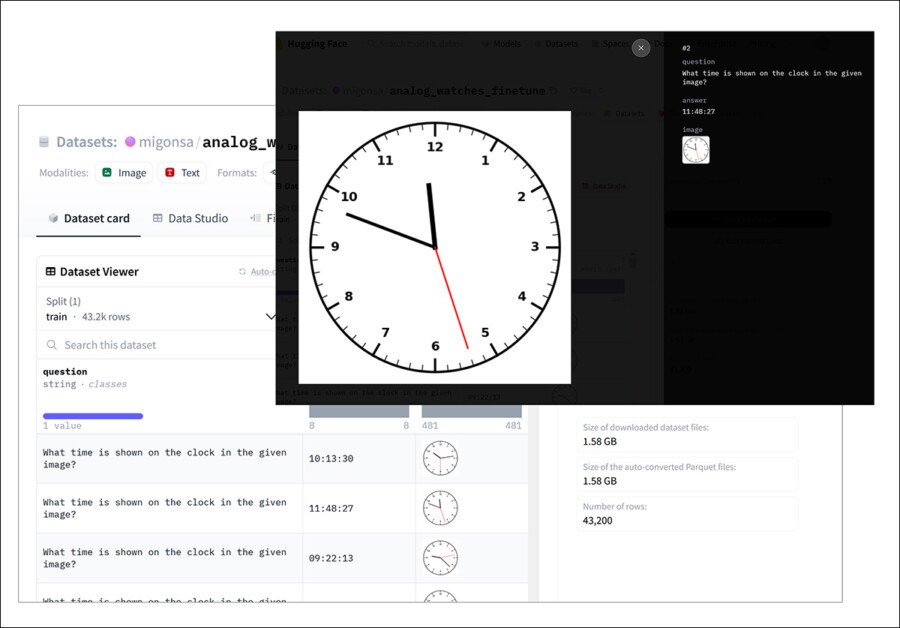
An instance from the researchers’ artificial analog clock dataset, used to fine-tune a GPT mannequin within the new work. Supply: https://huggingface.co/datasets/migonsa/analog_watches_finetune
Earlier than fine-tuning on the brand new dataset, GPT-4.1 persistently did not learn these clocks. After some publicity to the brand new assortment, nonetheless, its efficiency improved – however solely when the brand new photographs regarded like ones it had already seen.
When the form of the clock or the type of the arms modified, accuracy fell sharply; even small tweaks, resembling thinner arms or arrowheads (rightmost picture beneath), have been sufficient to throw it off; and GPT-4.1 struggled moreover to interpret Dali-esque ‘melting clocks’:
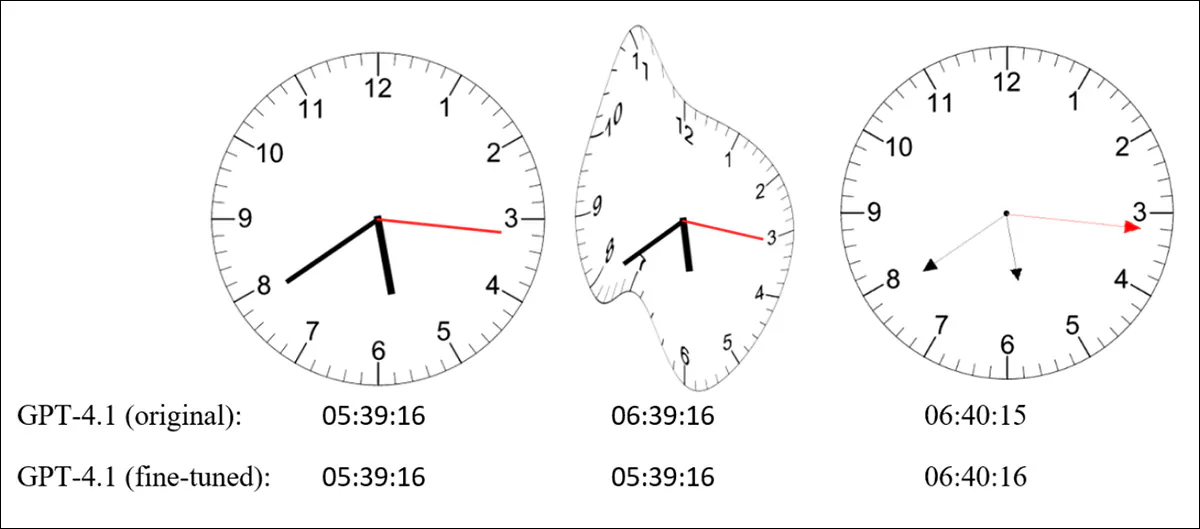
Clock photographs with normal design (left), distorted form (center), and modified arms (proper), alongside the instances returned by GPT-4.1 earlier than and after fine-tuning. Supply: https://arxiv.org/pdf/2505.10862
The authors deduce that present fashions resembling GPT-4.1 might subsequently be studying clock-reading primarily by visible sample matching, quite than any deeper idea of time, asserting:
‘[GPT 4.1] fails when the clock is deformed or when the arms are modified to be thinner and to have an arrowhead. The Imply Absolute Error (MAE) within the time estimate over 150 random instances was 232.48s for the preliminary clocks, 1380.69s when the form is deformed and 3726.93s when arms are modified.
‘These outcomes recommend that the MLLM has not realized to inform the time however quite memorized patterns.’
Sufficient Time
Most coaching datasets depend on scraped internet photographs, which are inclined to repeat sure instances – particularly 10:10, a widespread setting in watch ads:
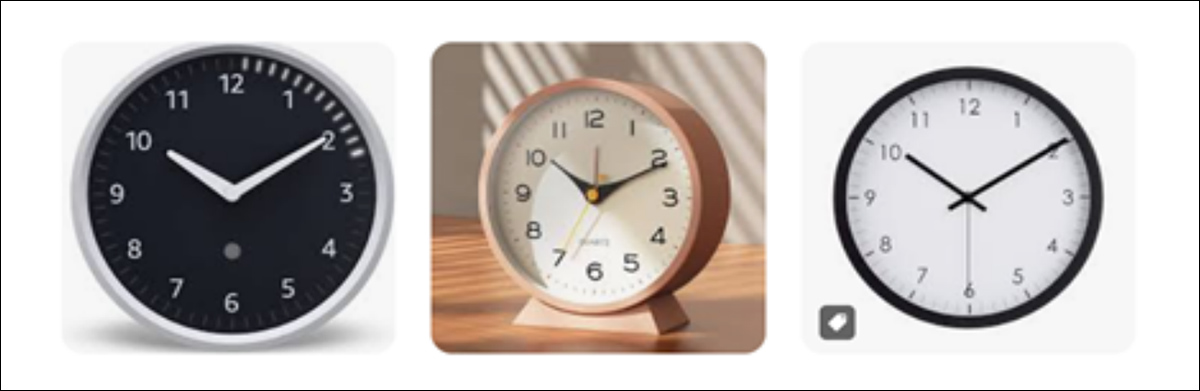
From the brand new paper, an instance of the prevalence of the ‘ten previous ten’ time in analog clock photographs.
Because of this restricted vary of instances depicted, the mannequin might even see solely a slim vary of doable clock configurations, limiting its capacity to generalize past these repetitive patterns.
Concerning why fashions fail to appropriately interpret the distorted clocks, the paper states:
‘Though GPT-4.1 performs exceptionally nicely with normal clock photographs, it’s shocking that modifying the clock arms by making them thinner and including arrowheads results in a major drop in its accuracy.
‘Intuitively, one would possibly count on that the extra visually complicated change – a distorted dial –would have a larger influence on efficiency, but this modification appears to have a comparatively smaller impact.
‘This raises a query: how do MLLMs interpret clocks, and why do they fail? One risk is that thinner arms impair the mannequin’s capacity to understand course, weakening its understanding of spatial orientation.
‘Alternatively, there could possibly be different components that trigger confusion when the mannequin makes an attempt to mix the hour, minute, and second arms into an correct time studying.’
The authors contend that figuring out the foundation trigger of those failures is vital to advancing multimodal fashions: if the problem lies in how the mannequin perceives spatial course, fine-tuning might provide a easy repair; but when the issue stems from a broader issue in integrating a number of visible cues, it factors to a extra basic weak spot in how these methods course of data.
Nice-Tuning Assessments
To check whether or not the mannequin’s failures could possibly be overcome with publicity, GPT-4.1 was fine-tuned on the aforementioned and complete artificial dataset. Earlier than fine-tuning, its predictions have been extensively scattered, with important errors throughout all forms of clocks. After fine-tuning on the gathering, accuracy improved sharply on normal clock faces, and, to a lesser extent, on distorted ones.
Nevertheless, clocks with modified arms, resembling thinner shapes or arrowheads, continued to supply massive errors.
Two distinct failure modes emerged: on regular and distorted clocks, the mannequin sometimes misjudged the course of the arms; however on clocks with altered hand types, it typically confused the operate of every hand, mistaking hour for minute or minute for second.
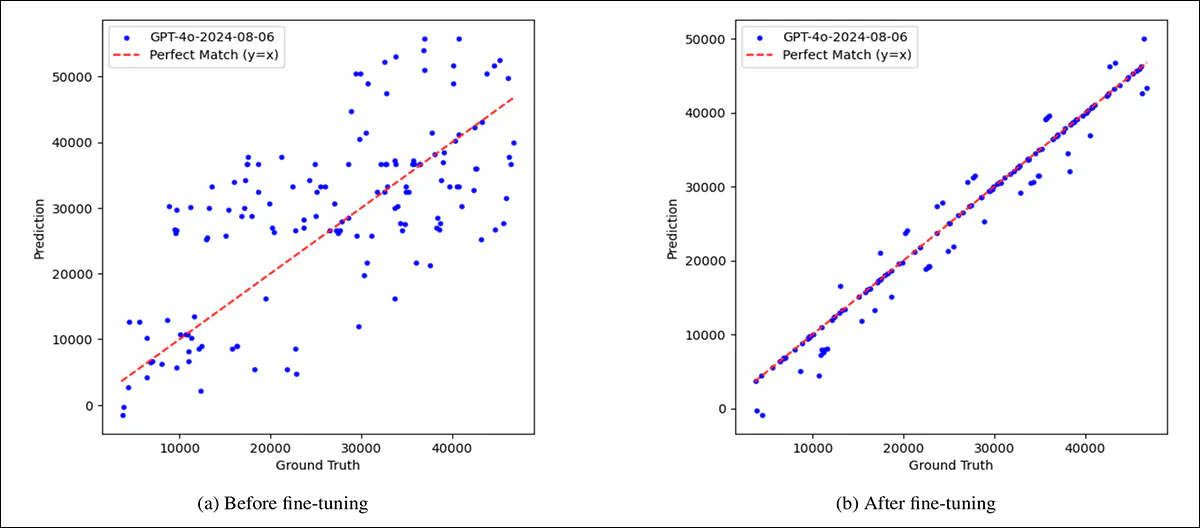
A comparability illustrating the mannequin’s preliminary weak spot, and the partial features achieved by fine-tuning, exhibiting predicted vs. precise time, in seconds, for 150 randomly chosen clocks. On the left, earlier than fine-tuning, GPT-4.1’s predictions are scattered and infrequently removed from the proper values, indicated by the pink diagonal line. On the fitting, after fine-tuning on a balanced artificial dataset, the predictions align rather more carefully with the bottom fact, though some errors stay.
This implies that the mannequin had realized to affiliate visible options like hand thickness with particular roles, and struggled when these cues modified.
The restricted enchancment on unfamiliar designs raises additional doubts about whether or not a mannequin of this sort learns the summary idea of time-telling, or merely refines its pattern-matching.
Hand Indicators
So, though fine-tuning improved GPT-4.1’s efficiency on standard analog clocks, it had far much less influence on clocks with thinner arms or arrowhead shapes, elevating the chance that the mannequin’s failures stemmed much less from summary reasoning and extra from confusion over which hand was which.
To check whether or not accuracy would possibly enhance if that confusion have been eliminated, a brand new evaluation was carried out on the mannequin’s predictions for the ‘modified-hand’ dataset. The outputs have been divided into two teams: circumstances the place GPT-4.1 appropriately acknowledged the hour, minute, and second arms; and circumstances the place it didn’t.
The predictions have been evaluated for Imply Absolute Error (MAE) earlier than and after fine-tuning, and the outcomes in comparison with these from normal clocks; angular error was additionally measured for every hand utilizing dial place as a baseline:

Error comparability for clocks with and with out hand-role confusion within the modified-hand dataset earlier than and after fine-tuning.
Complicated the roles of the clock arms led to the biggest errors. When GPT-4.1 mistook the hour hand for the minute hand or vice versa, the ensuing time estimates have been typically far off. In distinction, errors brought on by misjudging the course of a appropriately recognized hand have been smaller. Among the many three arms, the hour hand confirmed the best angular error earlier than fine-tuning, whereas the second hand confirmed the bottom.
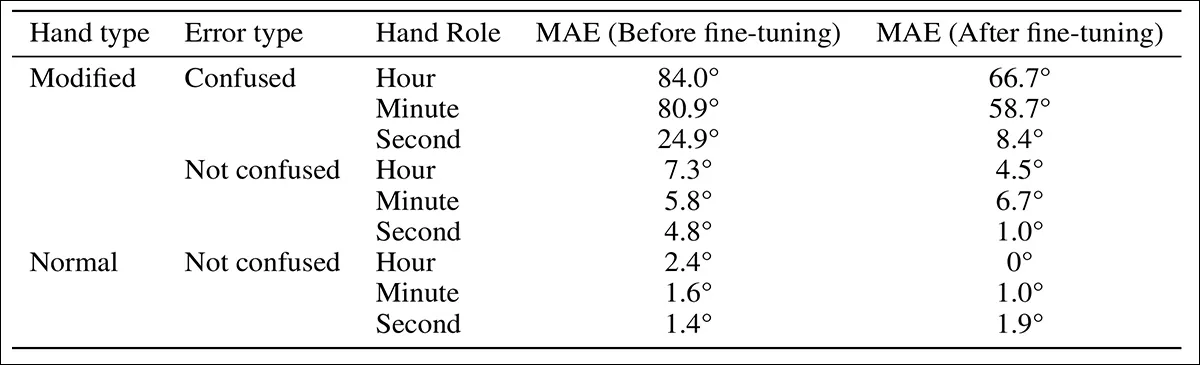
Angular error by hand kind for predictions with and with out hand-role confusion, earlier than and after fine-tuning, within the modified-hand dataset.
To deal with directional errors alone, the evaluation was restricted to circumstances the place the mannequin appropriately recognized every hand’s operate. If the mannequin had internalized a basic idea of time-telling, its efficiency on these examples ought to have matched its accuracy on normal clocks. It didn’t, and accuracy remained noticeably worse.
To look at whether or not hand form interfered with the mannequin’s sense of course, a second experiment was run: two new datasets have been created, every containing sixty artificial clocks with solely an hour hand, pointing to a unique minute mark. One set used the unique hand design, and the opposite the altered model. The mannequin was requested to call the tick mark that the hand was pointing to.
Outcomes confirmed a slight drop in accuracy with the modified arms, however not sufficient to account for the mannequin’s broader failures. A single unfamiliar visible characteristic appeared able to disrupting the mannequin’s total interpretation, even in duties it had beforehand carried out nicely.
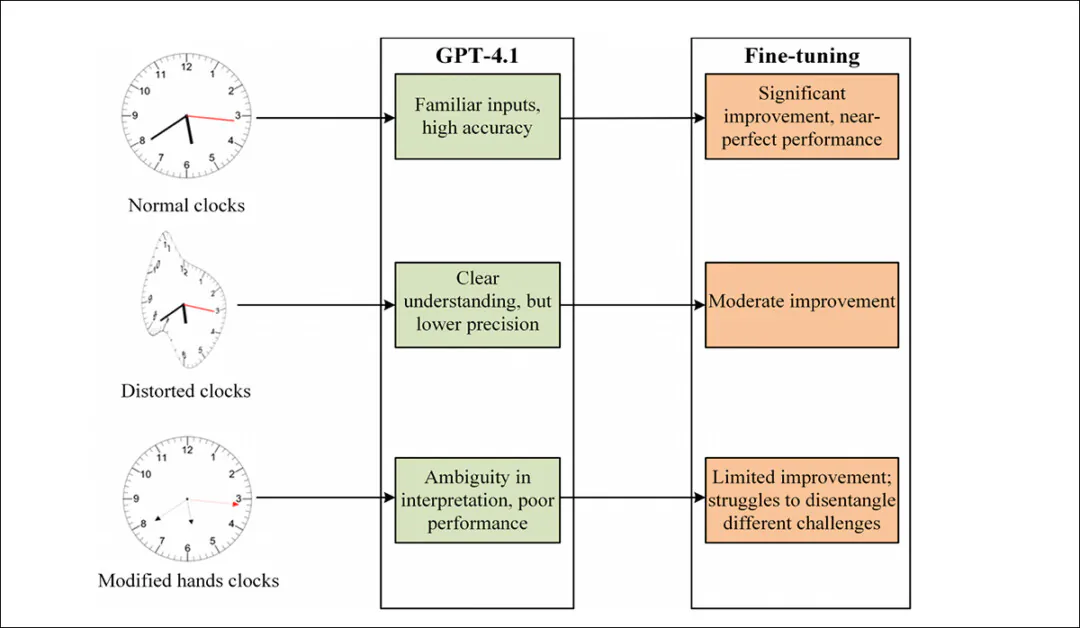
Overview of GPT-4.1’s efficiency earlier than and after fine-tuning throughout normal, distorted, and modified-hand clocks, highlighting uneven features and protracted weaknesses.
Conclusion
Whereas the paper’s focus could appear trivial at first look, it doesn’t particularly matter if vision-language fashions ever study to learn analog clocks at 100% accuracy. What offers the work weight is its deal with a deeper recurring query: whether or not saturating fashions with extra (and extra various) knowledge can result in the type of area understanding people purchase by abstraction and generalization; or whether or not the one viable path is to flood the area with sufficient examples to anticipate each doubtless variation at inference.
Both route raises doubts about what present architectures are really able to studying.
First printed Monday, Might 19, 2025