Introduction—financial revolution requires world methods, not level options
Editor’s be aware: This report is offered in partnership with Dell Applied sciences. Click on right here for extra info.
Analysis of synthetic intelligence (AI) successfully started with Alan Turing within the early Forties, and slowly progressed in suits and begins till the deep studying revolution of the 2010s. This period of analysis, pushed by teachers—and shortly after by {industry}—propelled the area ahead to the generative period we’re in right this moment. As corporations and nations right this moment open up their battle chests and spend trillions of {dollars} to advance AI capabilities, the long-term aim is synthetic common intelligence (AGI), however the short-term aim is incremental income positive factors that may fund the continued deployment of huge AI infrastructure tasks.
The state-of-play on this planet of AI suggests perception amongst captains of {industry} that each the short-term and long-term promise of AI will outcome finally in viable, sustainable enterprise circumstances; essentially, these enterprise circumstances might want to add trillions in income and world GDP to justify the huge funding in it—primarily deploy capital right this moment as a result of an financial revolution is coming. When that revolution is coming, nonetheless, is a troublesome query to reply.
Within the 2022 ebook “Energy and Prediction: The Disruptive Economics of Synthetic Intelligence,” authors and economists Ajay Agrawal, Joshua Gans and Avi Goldfarb describe “the between instances” whereby we’ve “witness[ed] the ability of this expertise and earlier than its widespread adoption.” The excellence is round AI as a degree resolution, “a easy substitute of older machine-generated predictive analytics with new AI instruments,” versus AI as a system able to essentially disrupting corporations, economies and industries.
The authors rightly draw an analog with electrical energy. Initially electrical energy served as a substitute to steam energy—a degree resolution. Over time electrical energy turned extra deeply embedded in manufacturing resulting in the bodily overhaul of how factories had been designed and operated; assume the reconfigurable meeting line—a brand new system. “These methods modifications altered the economic panorama,” the authors wrote. “Solely then did electrification lastly present up within the productiveness statistics, and in a giant method.”
So how does AI go from level resolution to system, and the way do these new methods scale globally? Know-how is clearly a key driver, however it’s essential to not overlook the established undeniable fact that system-level modifications embrace restructuring, each bodily and from a expertise perspective, how organizations conduct day-to-day enterprise. Setting apart how the expertise works, “The first advantage of AI is that it decouples prediction from the organizational design by way of reimagin[ing] how choices interrelate with each other.”
Utilizing AI to automate decision-making methods can be actually disruptive, and the capital outlays from the likes of Google, OpenAI and others align, with the assumption that these methods will usher in an AI-powered financial revolution. However when and the way AI has its electrical energy second is an open debate. “Simply as electrical energy’s true potential was solely unleashed when the broader advantages of distributed energy technology had been understood and exploited, AI will solely attain its true potential when its advantages in offering prediction will be totally leveraged,” Agrawal, Gans and Goldfarb wrote.
AI, they continued, “will not be solely in regards to the technical problem of accumulating knowledge, constructing fashions, and producing predictions, but in addition in regards to the organizational problem of enabling the proper people to make the proper choices on the proper time…The true transformation will solely come when innovators flip their consideration to creating new system options.”
1 | AI infrastructure—setting the stage, understanding the chance
Key takeaways:
- AI infrastructure is an interdependent ecosystem—from knowledge platforms to knowledge facilities and reminiscence to fashions, AI infrastructure is a synergistic area rife with disruptors.
- AI is evolving quickly—pushed by developments in {hardware}, entry to very large datasets and continuous algorithmic enhancements, gen AI is advancing at a fast tempo.
- All of it begins with the information—a typical AI workflow begins with knowledge preparation, a significant step in making certain the ultimate product delivers correct outputs at scale.
- AI is driving {industry} transformation and financial development—in just about each sector of the worldwide economic system, AI is enhancing effectivity, automating duties and turning predictive analytics into improved decision-making.
The AI revolution is driving unprecedented investments in digital and bodily infrastructure—spanning knowledge, compute, networking, and software program—to allow scalable, environment friendly, and transformative AI purposes. As AI adoption accelerates, enterprises, governments, and industries are constructing AI infrastructure to unlock new ranges of productiveness, innovation, and financial development.
Given the huge ecosystem of applied sciences concerned, this report takes a holistic view of AI infrastructure by defining its key elements and exploring its evolution, present impression, and future course.
For the needs of this report, AI infrastructure contains six core domains:
- Knowledge platforms – encompassing knowledge integration, governance, administration, and orchestration options that put together and optimize datasets for AI coaching and inference. Excessive-quality, well-structured knowledge is important for efficient AI efficiency.
- AI fashions – the multi-modal, open and closed algorithmic frameworks that analyze knowledge, acknowledge patterns, and generate outputs. These fashions vary from conventional machine studying (ML) to state-of-the-art generative AI and huge language fashions (LLMs).
- Knowledge heart {hardware} – contains high-performance computing (HPC) clusters, GPUs, TPUs, cooling methods, and energy-efficient designs that present the computational energy required for AI workloads.
- Networking – the fiber, Ethernet, wi-fi, and low-latency interconnects that transport huge datasets between distributed compute environments, from hyperscale cloud to edge AI deployments.
- Semiconductors – the specialised CPUs, GPUs, NPUs, TPUs, and customized AI accelerators that energy deep studying, generative AI, and real-time inference throughout cloud and edge environments.
- Reminiscence and storage – high-speed HBM (Excessive Bandwidth Reminiscence), DDR5, NVMe storage, and AI-optimized knowledge methods that allow environment friendly retrieval and processing of AI mannequin knowledge at totally different levels—coaching, fine-tuning, and inference.
And whereas these domains are distinct, AI infrastructure will not be a group of remoted applied sciences however reasonably an interdependent ecosystem the place every element performs a vital position in enabling scalable, environment friendly AI purposes. Knowledge platforms function the muse, making certain that AI fashions are skilled on high-quality, well-structured datasets. These fashions require high-performance computing (HPC) architectures in knowledge facilities, geared up with AI-optimized semiconductors similar to GPUs and TPUs to speed up coaching and inference. Nevertheless, processing energy alone will not be sufficient—quick, dependable networking is important to maneuver huge datasets between distributed compute environments, whether or not in cloud clusters, edge deployments, or on-device AI methods. In the meantime, reminiscence and storage options present the high-speed entry wanted to deal with the huge volumes of knowledge required for real-time AI decision-making. With no tightly built-in AI infrastructure stack, efficiency bottlenecks emerge, limiting scalability, effectivity, and real-world impression.
Collectively, these domains kind the muse of contemporary AI methods, supporting all the things from enterprise automation and digital assistants to real-time generative AI and autonomous decision-making.
The evolution of AI—from early experiments to generative intelligence
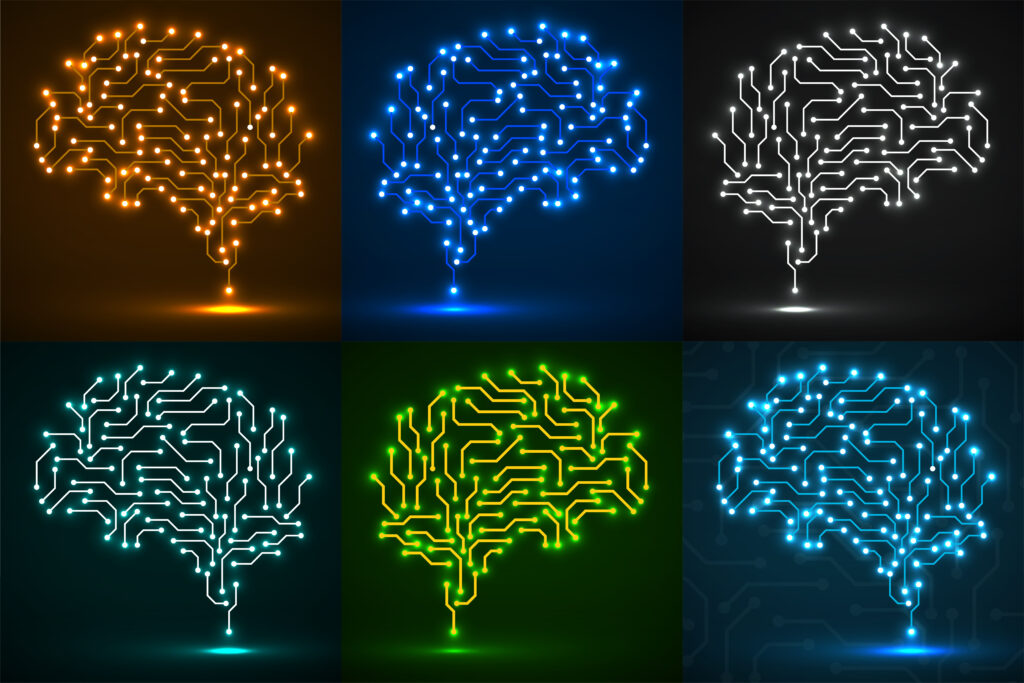
AI is usually described as a area of research centered on constructing laptop methods that may carry out duties requiring human-like intelligence. Whereas AI as an idea has been round because the Fifties, its early purposes had been largely restricted to rule-based methods utilized in gaming and easy decision-making duties.
A significant shift got here within the Eighties with machine studying (ML)—an method to AI that makes use of statistical methods to coach fashions from noticed knowledge. Early ML fashions relied on human-defined classifiers and have extractors, similar to linear regression or bag-of-words methods, which powered early AI purposes like electronic mail spam filters.
However because the world turned extra digitized—with smartphones, webcams, social media, and IoT sensors flooding the world with knowledge—AI confronted a brand new problem: the best way to extract helpful insights from this huge, unstructured info.
This set the stage for the deep studying breakthroughs of the 2010s, fueled by three key elements:
- Developments in {hardware}, significantly GPUs able to accelerating AI workloads
- The provision of huge datasets, vital for coaching highly effective fashions
- Enhancements in coaching algorithms, which enabled neural networks to mechanically extract options from uncooked knowledge
Immediately, we’re within the period of generative AI (gen AI) and huge language fashions (LLMs), with AI methods that exhibit surprisingly human-like reasoning and creativity. Functions like chatbots, digital assistants, real-time translation, and AI-generated content material have moved AI past automation and into a brand new section of clever interplay.
The intricacies of deep studying—making the organic synthetic
As Geoffrey Hinton, a pioneer of deep studying, put it: “I’ve all the time been satisfied that the one technique to get synthetic intelligence to work is to do the computation in a method just like the human mind. That’s the aim I’ve been pursuing. We’re making progress, although we nonetheless have heaps to study how the mind really works.”
Deep studying mimics human intelligence by utilizing deep neural networks (DNNs). These networks are impressed by organic neurons whereby dendrites obtain indicators from different neurons, the cell physique then course of these indicators, and the axon transmits info to the subsequent neuron. Synthetic neurons work equally. Layers of synthetic neurons course of knowledge hierarchically, enabling AI to carry out picture recognition, pure language processing, and speech recognition with human-like accuracy.
For instance, in picture classification (e.g., distinguishing cats from canines), a convolutional neural community (CNN) like AlexNet can be used. Not like earlier ML methods, deep studying doesn’t require handbook characteristic extraction—as a substitute, it mechanically learns patterns from knowledge.
A typical AI workflow—from knowledge to deployment
AI resolution improvement isn’t a single-step course of. It follows a structured workflow—often known as a machine studying or knowledge science workflow—which ensures that AI tasks are systematic, well-documented, and optimized for real-world purposes.
NVIDIA laid out 4 basic steps in an AI workflow:
- Knowledge preparation—each AI venture begins with knowledge. Uncooked knowledge should be collected, cleaned, and pre-processed to make it appropriate for coaching AI fashions. The scale of datasets utilized in AI coaching can vary from small structured knowledge to huge datasets with billions of parameters. However dimension alone isn’t all the things. NVIDIA emphasizes that knowledge high quality, variety, and relevance are simply as vital as dataset dimension.
- Mannequin coaching–as soon as knowledge is ready, it’s fed right into a machine studying or deep studying mannequin to acknowledge patterns and relationships. Coaching an AI mannequin requires mathematical algorithms to course of knowledge over a number of iterations, a step that’s extraordinarily computationally intensive.
- Mannequin optimization–after coaching, the mannequin must be fine-tuned and optimized for accuracy and effectivity. That is an iterative course of, with changes made till the mannequin meets efficiency benchmarks.
- Mannequin deployment and inference–a skilled mannequin is deployed for inference, which means it’s used to make predictions, choices, or generate outputs when uncovered to new knowledge. Inference is the core of AI purposes, the place a mannequin’s means to ship real-time, significant insights defines its sensible success.
This end-to-end workflow ensures the AI resolution delivers correct, real-time insights whereas being effectively managed inside an enterprise infrastructure.
The transformational AI alternative
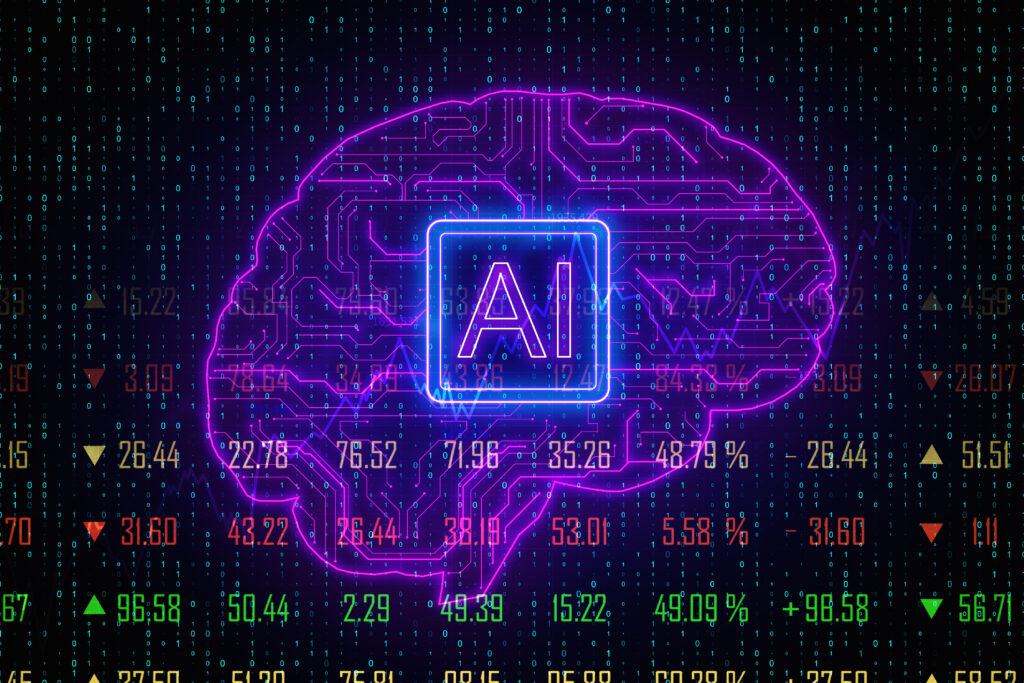
Picture courtesy of 123.RF.
“We’re leveraging the capabilities of AI to carry out intuitive duties on a scale that’s fairly arduous to think about. And no {industry} can afford or needs to overlook out on the massive benefit that predictive analytics affords.” That’s the message from NVIDIA co-founder and CEO Jensen Huang.
And based mostly on the sum of money being poured into AI infrastructure, Huang is correct. Whereas it’s nonetheless early days, industrial AI options are right this moment delivering tangible advantages largely based mostly on automation of enterprise processes and workflows. NVIDIA calls out AI’s means to boost effectivity, resolution making and organizational means for innovation by pulling out actionable, precious insights from huge datasets.
The corporate offers high-level examples of AI use circumstances for particular features and verticals:
- In name facilities, AI can energy digital brokers, extract knowledge insights and conduct sentiment evaluation.
- For retail companies, AI imaginative and prescient methods can analyze site visitors traits, buyer counts and aisle occupancy.
- Producers can use AI for product design, manufacturing optimization, enhanced high quality management and decreasing waste.
Right here’s what that appears like in the actual world:
- Mercedes-Benz is utilizing AI-powered digital twins to simulate manufacturing amenities, decreasing setup time by 50%.
- Deutsche Financial institution is embedding monetary AI fashions (fin-formers) to detect early threat indicators in transactions.
- Pharmaceutical R&D leverages AI to speed up drug discovery, chopping down a 10-year, $2B course of to months of AI-powered simulations.
The purpose is that AI is reshaping industries by enhancing effectivity, automating duties, and unlocking insights from huge datasets. From cloud computing to knowledge facilities, workstations, and edge gadgets, it’s driving digital transformation at each degree. Throughout sectors like automotive, monetary companies, and healthcare, AI is enabling automation, predictive analytics, and extra clever decision-making.
As AI evolves from conventional strategies to machine studying, deep studying, and now generative AI, its capabilities proceed to increase. Generative AI is especially transformative, producing all the things from textual content and pictures to music and video, demonstrating that AI will not be solely analytical but in addition inventive. Underpinning these developments is GPU acceleration, which has made deep studying breakthroughs doable and enabled real-time AI purposes.
Not simply an experimental expertise, AI is now a foundational device shaping the way in which companies function and innovate. There are many numbers on the market making an attempt to encapsulate the financial impression of AI; most all are within the trillions of {dollars}. McKinsey & Firm analyzed 63 gen AI use circumstances and pegged the annual financial contribution at $2.6 trillion to $4.4 trillion.
Ultimate thought: AI is not only a device—it’s turning into the spine of digital transformation, driving industry-wide disruption and financial development. However AI’s impression isn’t nearly smarter fashions or quicker chips—it’s in regards to the interconnected infrastructure that permits scalable, environment friendly, and clever methods.
2 | The AI infrastructure growth and coming financial revolution
Key takeaways:
- AI infrastructure funding is skyrocketing—the push to AGI, in addition to extra near-term adoption, is resulting in lots of of billions of capital outlay from massive tech.
- AI scaling legal guidelines are shaping AI infrastructure funding—provided that AI system efficiency is ruled by empirical scaling legal guidelines, together with rising scaling legal guidelines, all indicators level to the necessity for extra AI infrastructure to enhance AI outcomes and scale methods.
- Energy, not simply semiconductors, is a bottleneck—though the acute semiconductor scarcity has eased (however not disappeared), a doubtlessly greater bottleneck to AI infrastructure operationalization is entry to energy.
- The AI revolution might mirror previous financial transformations—historic precedents just like the agricultural and industrial revolutions recommend that AI-driven financial productiveness positive factors might result in important world financial development, however provided that infrastructure constraints will be managed successfully.
Within the sweep of all issues AI and attendant AI infrastructure investments, the aim, usually talking, is two-fold: incrementally leverage AI to ship monetizable, near-term capabilities that ship clear worth to customers and enterprises, whereas concurrently chipping away at reaching synthetic common intelligence (AGI), an ill-defined set of capabilities that might arguably deliver penalties that the world, collectively, might not be prepared for. The 2 complementary paths demand huge capital funding in AI infrastructure—each digital and bodily.
OpenAI CEO Sam Altman just lately posted a weblog that reiterated the corporate’s unequivocal dedication to “make sure that AGI…advantages all of humanity.” In exploring the trail to AGI, Altman delineated “three observations in regards to the economics of AI”:
- “The intelligence of an AI mannequin roughly equals the log of the assets used to coach and run it.”
- “The associated fee to make use of a given degree of AI falls about 10x each 12 months, and decrease costs result in far more use.” Extra right here on Jevons’ paradox.
- And “The socioeconomic worth of linearly growing intelligence is super-exponential in nature. A consequence of that is that we see no purpose for exponentially growing funding to cease within the close to future.”
Right here Altman is actually highlighting the AI scaling legal guidelines—greater datasets create greater fashions, necessitating better funding in AI infrastructure. This makes the case for corporations and nations to proceed spending lots of of billions—if not trillions—on AI capabilities that, in flip, ship outsized returns.
The race towards AGI and the continued enlargement of AI capabilities have led to a basic reality: greater fashions require extra knowledge and extra compute. This actuality is ruled by AI scaling legal guidelines—which clarify why AI infrastructure spending is rising exponentially.
A more in-depth have a look at AI scaling legal guidelines

Determine from “Scaling Legal guidelines for Neural Language Fashions”.
In January 2020, a staff of OpenAI researchers led by Jared Kaplan, who moved on to co-found Anthropic, revealed a paper titled “Scaling Legal guidelines for Neural Language Fashions.” The researchers noticed “exact power-law scalings for efficiency as a operate of coaching time, context size, dataset dimension, mannequin dimension and compute funds.” Primarily, the efficiency of an AI mannequin improves as a operate of accelerating scale in mannequin dimension, dataset dimension and compute energy. Whereas the industrial trajectory of AI has materially modified since 2020, the scaling legal guidelines proceed to be steadfast; and this has materials implications for the AI infrastructure that underlies the mannequin coaching and inference that customers more and more rely on.
Let’s break that down:
- Mannequin dimension scaling reveals that growing the variety of parameters in a mannequin usually improves its means to be taught and generalize, assuming it’s skilled on a ample quantity of knowledge. Enhancements can plateau if dataset dimension and compute assets aren’t proportionately scaled.
- Dataset dimension scaling relates mannequin efficiency to the amount and high quality of knowledge used for coaching. The significance of dataset dimension can diminish if mannequin dimension and compute assets aren’t proportionately scaled.
- Compute scaling mainly means extra compute (GPUs, servers, networking, reminiscence, energy, and many others…) equates to improved mannequin efficiency as a result of coaching can go on for longer, talking on to the wanted AI infrastructure.
In sum, a big mannequin wants a big dataset to work successfully. Coaching on a big dataset requires important funding in compute assets. Scaling considered one of these variables with out the others can result in course of and end result inefficiencies. Vital to notice right here the Chinchilla Scaling Speculation, developed by researchers at DeepMind and memorialized within the 2022 paper “Coaching Compute-Optimum Massive Language Fashions,” that claims scaling dataset and compute collectively will be more practical than constructing an even bigger mannequin.
“I’m a giant believer in scaling legal guidelines,” Microsoft CEO Satya Nadella stated in a current interview with Brad Gerstner and Invoice Gurley. He stated the corporate realized in 2017 “don’t wager in opposition to scaling legal guidelines however be grounded on exponentials of scaling legal guidelines turning into more durable. Because the [AI compute] clusters change into more durable, the distributed computing drawback of doing giant scale coaching turns into more durable.” long-term capex related to AI infrastructure deployment, Nadella stated, “That is the place being a hyperscaler I feel is structurally tremendous useful. In some sense, we’ve been practising this for a very long time.” He stated construct out prices will normalize, “then it will likely be you simply continue to grow just like the cloud has grown.”
Nadella defined within the interview that his present scaling constraints had been not round entry to the GPUs used to coach AI fashions however, reasonably, the ability wanted to run the AI infrastructure used for coaching.
And two extra AI scaling legal guidelines

Picture courtesy of NVIDIA.
Past the three AI scaling legal guidelines outlined above, Huang is monitoring two extra which have “now emerged.” These are the post-training scaling legislation and test-time scaling. Put up-training scaling refers to a sequence of methods used to enhance AI mannequin outcomes and make the methods extra environment friendly. A few of the related methods embrace:
- Superb-tuning a mannequin by including in domain-specific knowledge, successfully decreasing compute and knowledge required in comparison with constructing a brand new mannequin.
- Quantization reduces mannequin precision weights to make it smaller and quicker whereas sustaining acceptable efficiency and decreasing reminiscence and compute.
- Pruning removes pointless parameters in a skilled mannequin making it extra environment friendly with out efficiency decreases.
- Distillation primarily compresses information from a big mannequin to a small mannequin whereas retaining most capabilities.
- Switch studying re-uses a pre-trained mannequin for associated duties which means the brand new duties require much less knowledge and compute.
Huang likened post-training scaling to “having a mentor or having a coach offer you suggestions after you’re carried out going to high school. And so that you get exams, you get suggestions, you enhance your self.” That stated, “Put up-training requires an infinite quantity of computation, however the finish outcome produces unimaginable fashions.”
The opposite rising AI scaling legislation is test-time scaling which refers to methods utilized after coaching and through inference meant to boost efficiency and drive effectivity with out retraining the mannequin. A few of the core ideas listed below are:
- Dynamic mannequin adjustment based mostly on the enter or system constraints to steadiness accuracy and effectivity on the fly.
- Ensembling at inference combines predictions from a number of fashions or mannequin model to enhance accuracy.
- Enter-specific scaling adjusts mannequin habits based mostly on inputs at test-time to cut back pointless computation whereas retaining adaptability when extra computation is required.
- Quantization at inference reduces precision to hurry up processing.
- Lively test-time adaptation permits for mannequin tuning in response to knowledge inputs.
- Environment friendly batch processing teams inputs to maximise throughput to reduce computation overhead.
As Huang put it, test-time scaling is, “Whenever you’re utilizing the AI, the AI has the power to now apply a distinct useful resource allocation. As an alternative of bettering its parameters, now it’s centered on deciding how a lot computation to make use of to supply the solutions it needs to supply.”
Regardless, he stated, whether or not it’s post-training or test-time scaling, “The quantity of computation that we’d like, after all, is unimaginable…Intelligence, after all, is probably the most precious asset that we have now, and it may be utilized to unravel a number of very difficult issues. And so, [the] scaling legal guidelines…[are] driving monumental demand for NVIDIA computing.”
The evolution of AI scaling legal guidelines—from the foundational trio recognized by OpenAI to the extra nuanced ideas of post-training and test-time scaling championed by NVIDIA—underscores the complexity and dynamism of contemporary AI. These legal guidelines not solely information researchers and practitioners in constructing higher fashions but in addition drive the design of the AI infrastructure wanted to maintain AI’s development.
The implications are clear: as AI methods scale, so too should the supporting AI infrastructure. From the provision of compute assets and energy to developments in optimization methods, the way forward for AI will rely on balancing innovation with sustainability. As Huang aptly famous, “Intelligence is probably the most precious asset,” and scaling legal guidelines will stay the roadmap to harnessing it effectively. The query isn’t simply how giant we are able to construct fashions, however how intelligently we are able to deploy and adapt them to unravel the world’s most urgent challenges.
Alphabet, Amazon, Meta, Microsoft earmark $315 billion for AI infrastructure in 2025
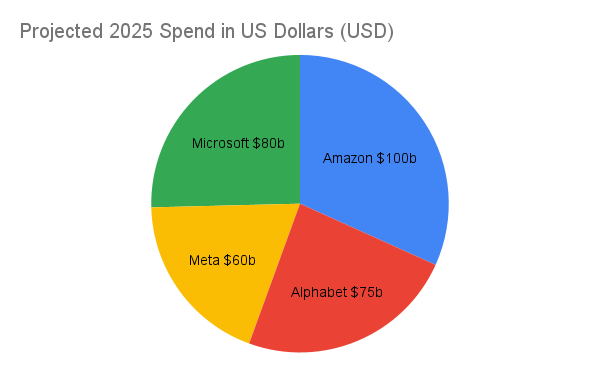
On condition that 4 of the largest corporations within the sport reported 2025 capex steering prior to now few weeks, we are able to put a quantity to deliberate AI infrastructure funding. For the needs of this evaluation, we’ll restrict capex steering to Alphabet (Google), Amazon, Meta and Microsoft. Within the coming 12 months, Amazon plans to spend $100 billion, Alphabet guided for $75 billion, Meta plans to speculate between $60 billion and $65 billion, and Microsoft expects to put out $80 billion. Between simply 4 U.S. tech giants, that’s $315 billion earmarked for AI infrastructure in 2025. And this doesn’t embrace huge investments popping out of China or the OpenAI-led Venture Stargate which is committing to $500 billion over 5 years.
The animating idea right here is that the speed of development in financial productiveness that the AI revolution might ship has historic parallels. Whereas these numbers are arduous to peg, if the change within the price of development of financial productiveness after the Agricultural Revolution and after the Industrial Revolution are instructive, a profitable AI revolution might result in extremely fast financial development. The extent of AI funding right this moment means that {industry} leaders anticipate a historic leap in financial productiveness. However how a lot development is feasible? Right here, historical past gives clues.
In his 2014 ebook Superintelligence, thinker Nick Bostrom attracts on a variety of knowledge to sketch out these revolutionary step modifications within the price of development of financial productiveness. In pre-history, he wrote, “development was so gradual that it took on the order of 1 million years for human productive capability to extend sufficiently to maintain an extra 1 million people dwelling at subsistence degree.” After the Agricultural Revolution, round 5000 BCE, “the speed of development had elevated to the purpose the place the identical quantity of development took simply two centuries. Immediately, following the Industrial Revolution, the world economic system grows on common by that quantity each 90 minutes.”
Drawing on modeling from economist Robin Hanson, financial and inhabitants knowledge suggests the “world economic system doubling time” was 224,000 years for early hunter gatherers. “For farming society, 909 years; and for industrial society, 6.3 years…If one other such transition to a distinct development mode had been to happen, and it had been of comparable magnitude to the earlier two, it will end in a brand new development regime through which the world economic system would double in dimension about each two weeks.” Whereas such a situation appears fantastical—and is constrained by AI infrastructure and energy provide—even a fraction of that development price can be transformative past comprehension.
Given the deliberate investments from hyperscalers, it’s clear that they purchase the narrative and see the ROIC. This additionally runs opposite to the rising discourse that we’re at present in an AI infrastructure funding bubble that can invariably burst leaving somebody to carry the bag. To wit: in contrast to previous speculative bubbles, AI infrastructure spending is instantly tied to scaling legal guidelines and quick enterprise demand and adoption, very similar to cloud computing investments within the 2010s that finally proved prescient.
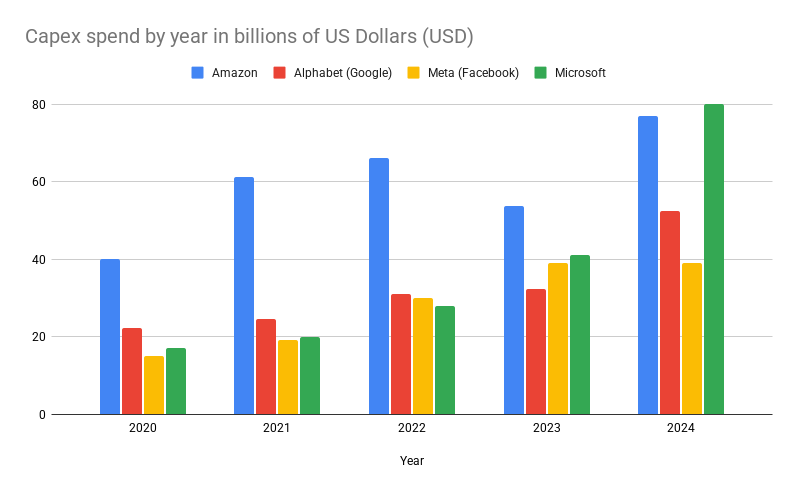
Reporting its fourth quarter and full-year 2024 financials on Feb. 4, Google-parent Alphabet CEO Sundar Pichai highlighted the corporate’s “differentiated full-stack method to AI innovation” of which its AI infrastructure is an important piece.
“Our refined world community of cloud areas and datacenters gives a robust basis for us and our clients, instantly driving income,” he stated, including that in 2024 Google “broke floor” on 11 new cloud areas and datacenter campuses. “Our main infrastructure can also be among the many world’s best. Google datacenters ship practically 4 instances extra computing energy per unit of electrical energy in comparison with simply 5 years in the past. These efficiencies, coupled with the scalability, price and efficiency we provide are why organizations more and more select Google Cloud’s platform.”
He continued: “I feel a number of it’s our power of the complete stack improvement, end-to-end optimization, our obsession with price per question…In case you have a look at the trajectory over the previous three years, the proportion of spend towards inference in comparison with coaching has been growing which is sweet as a result of, clearly, inferences to help companies with good ROIC…I feel that pattern is sweet.”
In sum, Pichai stated, “I feel a part of the rationale we’re so excited in regards to the AI alternative is, we all know we are able to drive extraordinary use circumstances as a result of the price of really utilizing it’s going to maintain coming down, which is able to make extra use circumstances possible. And that’s the chance area. It’s as massive because it comes, and that’s why you’re seeing us make investments to satisfy that second.”
Amazon is working to additional capitalize on what CEO Andy Jassy known as a “actually unusually giant, perhaps once-in-a-lifetime kind of alternative,” throughout an earnings name on Feb. 6. In a separate name with traders, Jassy pegged This fall capex at $26.3 billion and stated, “I feel that’s fairly consultant of what you anticipate [of] an annualized capex price in 2025…The overwhelming majority of that capex spend is on AI for AWS,” in accordance with reporting from CNBC.
Discussing how Amazon Internet Companies (AWS) is delivering AI-enabled companies, together with the underlying AI infrastructure all the way down to homegrown customized silicon for mannequin coaching and inference, Jassy stated, “AWS’s AI enterprise is a multi-billion greenback income run price enterprise that continues to develop at a triple-digit year-over-year share, and is rising greater than 3 times quicker at this stage of its evolution as AWS itself grew. And we felt like AWS grew fairly rapidly.”
Requested about demand for AI companies as in comparison with AWS’s capability to ship AI companies, Jassy stated he believes AWS has “extra demand that we might fulfill if we had much more capability right this moment. I feel just about everybody right this moment has much less capability than they’ve demand for, and it’s actually primarily chips which might be the realm the place corporations might use extra provide.”
“I really consider that the speed of development there has an opportunity to enhance over time as we have now greater and greater capability,” Jassy stated.
Reporting on its This fall 2024 on Jan. 29, Meta CEO Mark Zuckerberg highlighted adoption of the Meta AI private assistant, continued improvement of its Llama 4 LLM, and ongoing investments in AI infrastructure. “These are all massive investments,” he stated. “Particularly the lots of of billions of {dollars} that we are going to spend money on AI infrastructure over the long run. I introduced final week that we anticipate to deliver on-line virtually 1 [Gigawatt] of capability this 12 months, and we’re constructing a 2 [Gigawatt] and doubtlessly greater AI datacenter that’s so massive that it’ll cowl a big a part of Manhattan if it had been positioned there. We’re planning to fund all this by on the identical time investing aggressively in initiatives that use these AI advances to extend income development.”
CFO Susan Li stated This fall capex got here in at $14.8 billion “pushed by investments in servers, datacenters and community infrastructure. We’re working to satisfy the rising capability wants for these companies by each scaling our infrastructure footprint and growing the effectivity of our workloads. One other method we’re pursuing efficiencies is by extending the helpful lives of our servers and related networking tools. Our expectation going ahead is that we’ll be capable of use each our non-AI and AI servers for an extended time frame earlier than changing them, which we estimate can be roughly 5 and a half years.” She stated 2025 capex can be between $60 billion and $65 billion “pushed by elevated funding to help each our generative AI efforts and our core enterprise.”
And for Microsoft, Nadella stated, additionally on Jan. 29 earnings name, that enterprises “are starting to maneuver from proof-of-concepts to enterprise-wide deployments to unlock the complete ROI of AI.” The corporate’s AI enterprise handed an annual income run-rate of $13 billion, up 175% year-over-year in Q2 for fiscal 12 months 2025.
Nadella talked by means of the “core thesis behind our method to how we handle our fleet, and the way we allocate our capital to compute. AI scaling legal guidelines are persevering with to compound throughout each pre-training and inference-time compute. We ourselves have been seeing important effectivity positive factors in each coaching and inference for years now. On inference, we have now usually seen greater than 2X price-performance acquire for each {hardware} technology, and greater than 10X for each mannequin technology resulting from software program optimizations.”
He continued: “And, as AI turns into extra environment friendly and accessible, we’ll see exponentially extra demand. Subsequently, a lot as we have now carried out with the industrial cloud, we’re centered on constantly scaling our fleet globally and sustaining the proper steadiness throughout coaching and inference, in addition to geo distribution. Any more, it’s a extra steady cycle ruled by each income development and functionality development, because of the compounding results of software-driven AI scaling legal guidelines and Moore’s legislation.”
On datacenter funding, Nadella stated Microsoft’s Azure cloud is the “infrastructure layer for AI. We proceed to increase our datacenter capability according to each near-term and long-term demand indicators. Now we have greater than doubled our total datacenter capability within the final three years. And we have now added extra capability final 12 months than every other 12 months in our historical past. Our datacenters, networks, racks and silicon are all coming collectively as a whole system to drive new efficiencies to energy each the cloud workloads of right this moment and the next-gen AI workloads.”
Again to Altman: “Making certain that the advantages of AGI are broadly distributed is vital. The historic impression of technological progress means that many of the metrics we care about (well being outcomes, financial prosperity, and many others.) get higher on common and over the long-term, however growing equality doesn’t appear technologically decided and getting this proper could require new concepts.”
Ultimate thought: The dimensions of funding indicators that main gamers aren’t simply chasing a speculative future—they’re betting on AI as the subsequent basic driver of financial transformation. The following decade will reveal whether or not AI’s infrastructure spine can match the ambitions fueling its enlargement.
3 | Can knowledge heart deployment rise to satisfy the AI alternative?
Key takeaways:
- AI is remodeling knowledge heart funding and technique—for AI to ship on its promise of financial revolution, huge multi-trillion {dollars} investments in modernizing current knowledge facilities for AI and constructing new knowledge facilities particularly for AI.
- Each knowledge heart is turning into an AI knowledge heart—AFCOM discovered that 80% of knowledge heart operators are planning main capability will increase to maintain tempo with present and future demand for AI computing.
- AI-based energy demand is reshaping vitality methods—knowledge heart electrical energy consumption is anticipated to double within the coming years, driving a shift towards environment friendly {hardware}, optimized energy utilization, AI-driven vitality administration and different methods aimed toward making certain the enlargement of AI infrastructure doesn’t include a proportional enhance in vitality consumption.
- There’s a brand new hyperscaler coming to city—Venture Stargate is a $500 billion wager on AI infrastructure management, positioning SoftBank, MGX, OpenAI, and Oracle as a brand new hyperscaler, with 100,000-GPU clusters and 10+ knowledge facilities deliberate in Texas to safe U.S. dominance in AI.
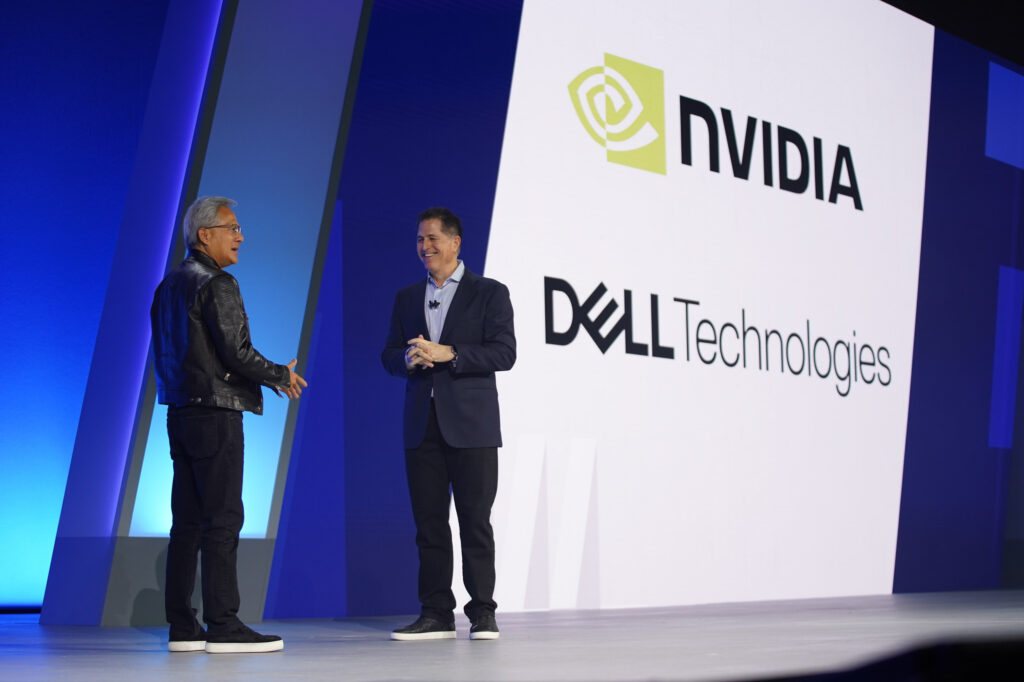
Earlier than waiting for what AI means for a way knowledge facilities are designed, deployed, operated and powered, let’s look again to lower than a 12 months in the past when ChatGPT had made its impression, however earlier than probably the most precious corporations on this planet allotted lots of of billions in capital to construct out the information heart, and complementary, infrastructure wanted for the AI revolution.
It was Dell Applied sciences World 2024, held yearly in Could. Firm founder and CEO Michael Dell took the stage and mirrored on factories of the previous the place the method was based mostly on turning a mill utilizing wind or water, then utilizing electrical energy to show the wheel then, finally, bringing electrical energy deeper into the method to automate duties with purpose-built machines–the evolution from a degree resolution to a system, primarily. “That’s sort of the place we are actually with AI,” Dell stated. However, he cautioned to keep away from the temptation to make use of AI to easily flip the figurative wheel; reasonably, the aim must be to reinvent the group with AI at its heart. “It’s a generational alternative for productiveness and development.”
Joined onstage by NVIDIA’s Huang, the pair mentioned the roughly $1 trillion in knowledge heart modernization, and orders of magnitude greater than that—the quantity $100 trillion was thrown out—in internet new knowledge heart capability that must be constructed to help the calls for of AI. Final phrases from Dell: “The true query isn’t how massive AI goes to be, however how a lot good is AI going to do? How a lot good can AI do for you?…Reinventi[ng] and reimagining your group is tough. It feels dangerous, even horrifying. However the greater threat, and what’s much more horrifying, is what occurs if you happen to don’t do it.”
Within the lower than a 12 months since Dell and NVIDIA made the case for quick, important investments into AI infrastructure, the message (which has been repeated again and again and over within the interim) was heard loud and clear by knowledge heart homeowners and operators. In January this 12 months, AFCOM, an expert affiliation for knowledge heart and IT infrastructure career, launched its annual “State of the Knowledge Middle” report. Leaping straight to the conclusion, AFCOM discovered that, “Each knowledge heart is turning into an AI knowledge heart.”
Primarily based on a survey of knowledge heart operators, AFCOM discovered that 80% of respondents anticipate “important will increase in capability necessities resulting from AI workloads. Almost 65% stated they’re actively deploying AI-capable options. Rack density has greater than doubled from 2021 to current, with practically 80% of respondents anticipating rack density can be pushed additional resulting from AI and high-performance workloads. Which means knowledge heart managers wish to enhance air stream, undertake liquid cooling and likewise use new sensors for monitoring.
To that energy level, AFCOM discovered that greater than 100 megawatts of latest knowledge heart development has been added each single month since late 2021; the truth is, the US colocation knowledge heart market has greater than doubled in dimension prior to now 4 years with file low emptiness charges and rents steadily growing. Photo voltaic vitality is seen as the important thing renewable vitality supply for knowledge facilities, in accordance with 55% of respondents, whereas curiosity in nuclear energy can also be on the rise.
“The information heart has developed from a supporting participant to the cornerstone of digital transformation. It’s the engine behind probably the most revolutionary options shaping our world right this moment,” stated Invoice Kleyman, who’s CEO and co-founder of Apolo.us and program chair for AFCOM’s Knowledge Middle World occasion. “This can be a time to embrace daring experimentation and collective innovation, push boundaries, undertake transformative applied sciences and deal with sustainability to organize for what lies forward.”
Kleyman additionally stated: “Up to now 12 months, our relationship with knowledge has shifted profoundly. This isn’t simply one other tech pattern; it’s a foundational change in how people interact with info.”
The vitality calls for of AI infrastructure
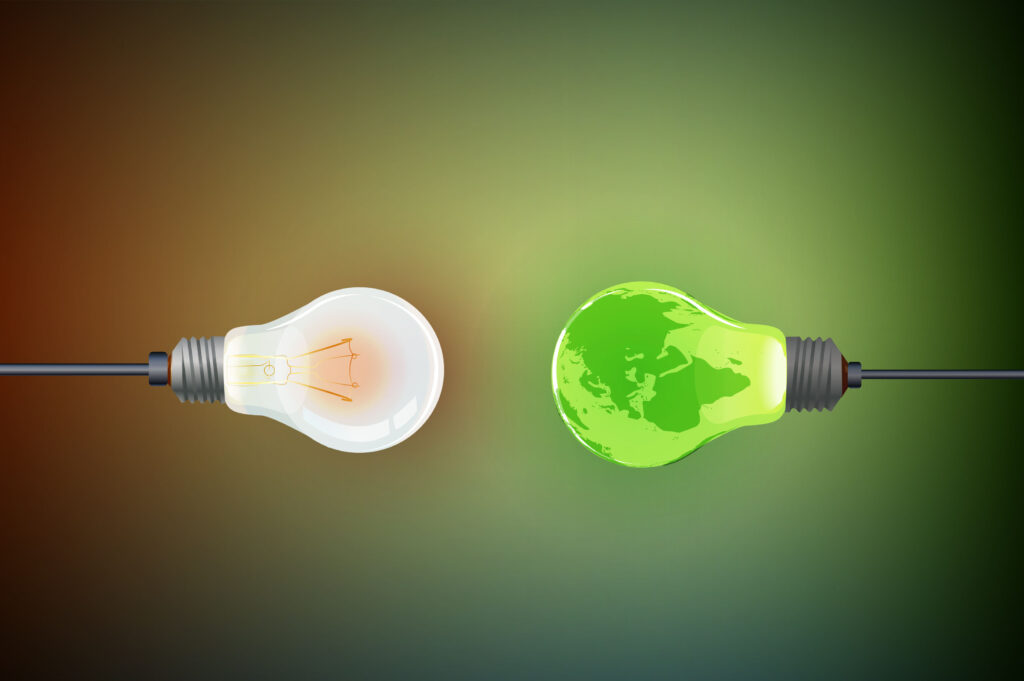
The fast development of AI and the investments fueling its enlargement have considerably intensified the ability calls for of knowledge facilities. Globally, knowledge facilities consumed an estimated 240–340 TWh of electrical energy in 2022—roughly 1% to 1.3% of world electrical energy use, in accordance with the Worldwide Vitality Company (IEA). Within the early 2010s, knowledge heart vitality footprints grew at a comparatively average tempo, because of effectivity positive factors and the shift towards hyperscale amenities, that are extra environment friendly than smaller server rooms.
That secure development sample has given technique to explosive demand. The IEA tasks that world knowledge heart electrical energy consumption might double between 2022 and 2026. Equally, IDC forecasts that surging AI workloads will drive an enormous enhance in knowledge heart capability and energy utilization, with world electrical energy consumption from knowledge facilities projected to double to 857 TWh between 2023 and 2028. AI-specific infrastructure is on the core of this development, with IDC estimating that AI knowledge heart capability will increase at a 40.5% CAGR by means of 2027.
Given the huge vitality necessities of right this moment’s knowledge facilities and the even better calls for of AI-focused infrastructure, stakeholders should implement methods to enhance vitality effectivity. This isn’t solely important for sustaining AI’s development but in addition aligns with the net-zero targets set by many corporations inside the AI infrastructure ecosystem. Key strategic pillars embrace:
- Upgrading to extra environment friendly {hardware} and architectures—generational enhancements in semiconductors, servers, and energy administration elements ship incremental positive factors in vitality effectivity. Optimizing workload scheduling may maximize server utilization, making certain fewer machines deal with extra work and minimizing idle energy consumption.
- Optimizing Energy Utilization Effectiveness (PUE)—PUE is a key metric for assessing knowledge heart effectivity by contemplating cooling, energy distribution, airflow, and different elements. Hyperscalers like Google have decreased PUE by implementing scorching/chilly aisle containment, elevating temperature setpoints, and investing in energy-efficient uninterruptible energy provide (UPS) methods.
- AI-driven administration and analytics—AI itself may help mitigate the vitality challenges it creates. AI-driven real-time monitoring and automation can dynamically regulate cooling, energy distribution, and useful resource allocation to optimize effectivity.
One of many major objectives is to scale AI infrastructure globally with out a proportional enhance in vitality consumption. Attaining this requires a mixture of funding in trendy {hardware}, developments in facility design, and AI-enhanced operational effectivity.
Liquid cooling and knowledge heart design
As rack densities enhance to help power-hungry GPUs and different AI accelerators, cooling is turning into a vital problem. The necessity for extra superior cooling options will not be solely shaping vendor competitors but in addition influencing knowledge heart web site choice, as operators think about local weather situations when designing new amenities.
Whereas liquid cooling will not be new, the rise of AI has intensified curiosity in direct-to-chip chilly plates and immersion cooling—applied sciences that take away warmth way more effectively than air cooling. In direct-to-chip cooling, liquid circulates by means of chilly plates connected to AI semiconductors, whereas immersion cooling submerges whole servers in a cooling fluid. Geography additionally performs a job, with some operators leveraging free cooling, which makes use of ambient air or evaporative cooling towers to dissipate warmth with minimal vitality consumption.
Whereas liquid and free cooling require increased upfront capital investments than conventional air cooling, the long-term advantages are substantial—together with decrease operational prices, improved vitality effectivity, and the power to help next-generation AI workloads.
New AI-focused knowledge facilities are being designed from the bottom as much as accommodate higher-density computing and superior cooling options. Key design diversifications embrace:
- Decrease rack density per room and elevated spacing to optimize airflow and liquid cooling effectivity.
- Enhanced flooring loading capability to help liquid cooling tools and accommodate heavier infrastructure.
- Larger-voltage DC energy methods to enhance vitality effectivity and combine with superior battery storage options.
Taken collectively, liquid cooling and knowledge heart design improvements can be essential for scaling AI infrastructure with out compromising vitality effectivity or sustainability.
The Stargate Venture—meet the most recent hyperscaler

Picture courtesy of OpenAI.
As detailed within the earlier part, main world tech corporations are poised to speculate lots of of billions into AI infrastructure in 2025, however the $500 billion Stargate Venture represents an unprecedented mobilization of capital. Framed as a strategic, long-term funding in U.S. technological management, Venture Stargate goals to place the nation on the forefront of the race towards AGI.
The lead traders in Stargate Venture are Japan’s SoftBank and UAE-based funding agency MGX. OpenAI, which would be the anchor tenant of the deliberate knowledge facilities, and Oracle are additionally throwing in fairness, though doubtless at a decrease degree than SoftBank and MGX. SoftBank is tasked with working issues on the monetary facet whereas OpenAI would be the lead operational companion. The $500 billion in capital is deliberate to be deployed over a four-year interval with $100 billion coming this 12 months.
The venture’s proponents body the spending as a strategic long-term wager. The return on funding is envisioned extra in geopolitical and financial phrases than quick revenue. SoftBank’s Chairman and CEO Masayoshi Son known as it “the start of our Golden Age,” indicating confidence that this can unlock new industries and large financial worth. OpenAI stated in an announcement that the Stargate Venture “will safe American management in AI, create lots of of hundreds of American jobs, and generate huge financial advantages for all the world.”
OpenAI particularly known as out its ambitions to leverage the brand new AI infrastructure funding to develop AGI “for the good thing about all of humanity. We consider that this new step is vital on the trail, and can allow inventive individuals to determine the best way to use AI to raise humanity.”
Along with the fairness companions, Stargate Venture has named a number of expertise companions, together with Arm (which is owned by SoftBank), Microsoft (an OpenAI investor and at present major supplier of knowledge heart capability), NVIDIA and Oracle. Along with being an fairness companion, Oracle has been constructing knowledge facilities in Texas which might be being folded into the primary section. Along with its cloud take care of Microsoft, OpenAI additionally has current preparations with Oracle for GPU capability.
Oracle’s pre-existing knowledge heart enlargement in Texas has now change into a core preliminary web site for Venture Stargate. Earlier than the venture’s announcement, Oracle had already begun deploying a 100,000-GPU cluster at its Abilene, Texas campus. Oracle co-founder and CTO Larry Ellison has acknowledged that the corporate is constructing 10 knowledge facilities in Texas, every 500,000 sq. toes, with plans to scale to twenty extra areas in future phases of Venture Stargate.
As Stargate’s first section accelerates, with extra particulars on future phases anticipated, one factor is already evident—this initiative successfully introduces a brand new hyperscaler into the AI infrastructure panorama. With unprecedented capital deployment, strategic partnerships, and a robust nationwide safety narrative, Stargate Venture is poised to reshape the aggressive dynamics of AI infrastructure and reinforce U.S. dominance within the race towards AGI.
Ultimate thought: AI is prompting an historic overhaul of knowledge heart infrastructure, however it’s nonetheless early innings. The query isn’t whether or not AI knowledge facilities will dominate, however who will management this next-generation infrastructure and the way they may steadiness innovation with sustainability.
4 | The convergence of test-time inference scaling and edge AI
Key takeaways:
- Check-time inference scaling is essential for AI effectivity—as AI execution shifts from centralized clouds to distributed edges, adjusting compute assets throughout inference can enhance effectivity, latency and efficiency.
- Edge AI is remodeling AI deployment—working inference regionally, both on-devices or on the edge, delivers advantages to the tip consumer whereas bettering system-level economics.
- The “reminiscence wall” is a possible bottleneck—AI chips can course of knowledge quicker than reminiscence methods can ship knowledge, however addressing this limitation to make edge AI is a precedence.
- Agentic AI will unlock real-time decision-making on the edge—AI brokers working regionally will autonomously flip knowledge into choices whereas constantly studying. Agentic AI on the edge will assist enterprise AI methods dynamically regulate to new inputs with out counting on costly, time-consuming mannequin re-training cycles.
As AI shifts from centralized clouds to distributed edge environments, the problem is not simply mannequin coaching—it’s scaling inference effectively. Check-time inference scaling is rising as a vital enabler of real-time AI execution, permitting AI fashions to dynamically regulate compute assets at inference time based mostly on activity complexity, latency wants, and obtainable {hardware}. This shift is fueling the fast rise of edge AI, the place fashions run regionally on gadgets as a substitute of counting on cloud knowledge facilities—bettering pace, privateness, and cost-efficiency.
Latest discussions have highlighted the significance of test-time scaling whereby AI methods can allocate assets dynamically, breaking down issues into a number of steps and evaluating varied responses to boost efficiency. This method is proving to be extremely efficient.
The emergence of test-time scaling methods has important implications for AI infrastructure, particularly regarding edge AI. Edge AI includes processing knowledge and working AI fashions regionally on gadgets or close to the information supply, reasonably than relying solely on centralized clouds. This method affords a number of benefits:
- Diminished latency: Processing knowledge nearer to its supply minimizes the time required for knowledge transmission, enabling quicker decision-making.
- Improved privateness: Native knowledge processing reduces the necessity to transmit delicate info to centralized servers, enhancing knowledge privateness and safety.
- Bandwidth effectivity: By dealing with knowledge regionally, edge AI reduces the demand on community bandwidth, which is especially helpful in environments with restricted connectivity. And, after all, decreasing community utilization for knowledge transport has a direct line to price.
And {industry} deal with edge AI is gaining traction. Qualcomm, as an example, has been discussing the subject for greater than two years, and sees edge AI as a big development space. In a current earnings name, Qualcomm CEO Cristiano Amon highlighted the growing demand for AI inference on the edge, viewing it as a “tailwind” for his or her enterprise.
“The period of AI inference”
Amon just lately informed CNBC’s Jon Fortt, “We began speaking about AI on the sting, or on gadgets, earlier than it was common.” Digging in additional on an earnings name, he stated, “Our superior connectivity, computing and edge AI applied sciences and product portfolio proceed to be extremely differentiated and more and more related to a broad vary of industries…We additionally stay very optimistic in regards to the rising edge AI alternative throughout our enterprise, significantly as we see the subsequent cycle of AI innovation and scale.”
Amon dubbed that subsequent cycle “the period of AI inference.” Past test-time inference scaling, this additionally aligns with different traits round mannequin dimension discount permitting them to run regionally on gadgets like handsets and PCs. “We anticipate that whereas coaching will proceed within the cloud, inference will run more and more on gadgets, making AI extra accessible, customizable and environment friendly,” Amon stated. “It will encourage the event of extra focused, purpose-oriented fashions and purposes, which we anticipate will drive elevated adoption, and in flip, demand for Qualcomm platforms throughout a variety of gadgets.”
Bottomline, he stated, “We’re well-positioned to drive this transition and profit from this upcoming inflection level.” Increasing on that time in dialog with Fortt, Amon stated the shifting AI panorama is “an ideal tailwind for enterprise and sort of materializes what we’ve been making ready for, which is designing chips that may run these fashions on the edge.”
Intel, which is struggling to distinguish its AI worth proposition and is popping focus to improvement of “rack-scale” options for AI datacenters, additionally sees edge AI as an rising alternative. Co-CEO Michelle Johnston Holthaus talked it out throughout a quarterly earnings name. AI “is a lovely marketplace for us over time, however I’m not proud of the place we’re right this moment,” she stated. “On the one hand, we have now a number one place because the host CPU for AI servers, and we proceed to see a big alternative for CPU-based inference on-prem and on the edge as AI-infused purposes proliferate. However, we’re not but taking part within the cloud-based AI datacenter market in a significant method.”
She continued: “AI will not be a market within the conventional sense. It’s an enabling utility that should span throughout the compute continuum from the datacenter to the sting. As such, a one-size-fits-all method is not going to work, and I can see clear alternatives to leverage our core belongings in new methods to drive probably the most compelling whole price of possession throughout the continuum.”
Holthaus’s, and Intel’s, view of edge AI inference as a development space pre-date her tenure as co-CEO. Former CEO Pat Gelsinger, talking at a CES keynote in 2024, made the case within the context of AI PCs and laid out the three legal guidelines of edge computing. “First is the legal guidelines of economics,” he stated on the time. “It’s cheaper to do it in your gadget…I’m not renting cloud servers…Second is the legal guidelines of physics. If I’ve to round-trip the information to the cloud and again, it’s not going to be as responsive as I can do regionally…And third is the legal guidelines of the land. Am I going to take my knowledge to the cloud or am I going to maintain it on my native gadget?”
Put up-Intel, Gelsinger has continued this space of focus with an funding in U.Ok.-based startup Fractile which makes a speciality of AI {hardware} that makes a speciality of in-memory inference reasonably than shifting mannequin weights from reminiscence to a processor. Writing on LinkedIn in regards to the funding, Gelsinger stated, “Inference of frontier AI fashions is bottlenecked by {hardware}. Even earlier than test-time compute scaling, price and latency had been big challenges for large-scale LLM deployments. With the appearance of reasoning fashions, which require memory-bound technology of hundreds of output tokens, the constraints of current {hardware} roadmaps [have] compounded. To realize our aspirations for AI, we’d like radically quicker, cheaper and far decrease energy inference.”
Verizon, in a transfer indicative of the bigger alternative for operators to leverage current distributed belongings in service of latest income from AI enablement, just lately launched the AI Join product suite. The corporate described the providing as “designed to allow companies to deploy…AI workloads at scale. Verizon highlighted McKinsey estimates that by 2030 60% to 70% of AI workloads can be “real-time inference…creating an pressing want for low-latency connectivity, compute and safety on the edge past present demand.” All through its community, Verizon has fiber, compute, area, energy and cooling that may help edge AI; Google Cloud and Meta are already utilizing a few of Verizon’s capability, the corporate stated.
Agentic AI on the edge
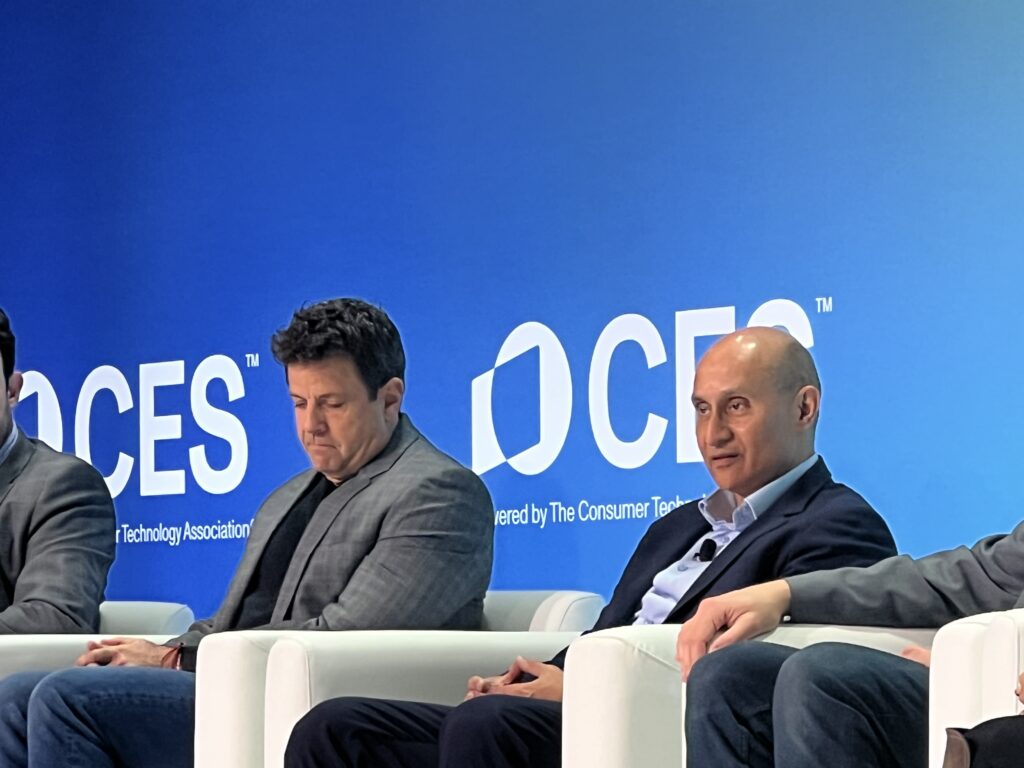
Qualcomm’s Durga Malladi mentioned edge AI at CES 2025.
Wanting additional out, Qualcomm’s Durga Malladi, talking throughout CES 2025, tied collectively agentic and edge AI. The thought is that on-device AI brokers will entry your apps in your behalf, connecting varied dots in service of your request and ship an end result not tied to at least one explicit utility. On this paradigm, the consumer interface of a wise telephone modifications; as he put it, “AI is the brand new UI.”
He tracked computing from command line interfaces to graphical interfaces accessible with a mouse. “Immediately we stay in an app-centric world…It’s a really tactile factor…The reality is that for the longest time frame, as people, we’ve been studying the language of computer systems.” AI modifications that; when the enter mechanism is one thing pure like your voice, the UI can now remodel utilizing AI to change into extra customized and private. “The front-end is dominated by an AI agent…that’s the transformation that we’re speaking of from a UI perspective.”
He additionally mentioned how an area AI agent will co-evolve with its consumer. “Over time there’s a private information graph that evolves. It defines you as you, not as another person.” Localized context, made doable by on-device AI, or edge AI extra broadly, will enhance agentic outcomes over time. “And that’s an area the place I feel, from the tech {industry} standpoint, we have now a number of work to do.”
Dell Applied sciences can also be taking a look at this intersection of agentic and edge AI. In an interview, Pierluca Chiodelli, vice chairman of engineering expertise, edge portfolio product administration and buyer operations, underscored this basic shift taking place in AI: companies are shifting away from a cloud-first mindset and embracing a hybrid AI mannequin that connects a continuum throughout gadgets, the sting and the cloud.
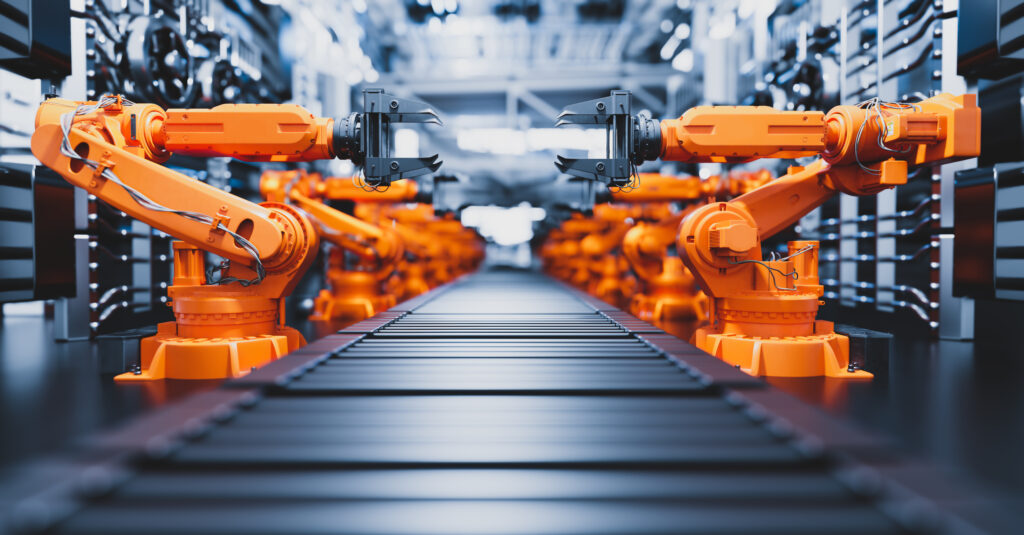
Picture courtesy of 123.RF.
Because it pertains to agentic AI methods working in edge environments, Chiodelli used laptop imaginative and prescient in manufacturing for instance. Immediately, high quality management AI fashions run inference on the edge—detecting defects, deviations, or inefficiencies in a manufacturing line. But when one thing sudden occurs, similar to a delicate shift in supplies or lighting situations, the mannequin can fail. The method to retrain the mannequin takes without end, Chiodelli defined. You must manually gather knowledge, ship it to the cloud or an information heart for retraining, then redeploy an up to date mannequin again to the sting. That’s a gradual, inefficient course of.
With agentic AI, as a substitute of counting on centralized retraining cycles, AI brokers on the edge might autonomously detect when a mannequin is failing, collaborate with different brokers, and proper the difficulty in actual time. “Agentic AI, it really means that you can have a bunch of brokers that work collectively to right issues.”
For industries that depend on precision, effectivity, and real-time adaptability, similar to manufacturing, healthcare, and vitality, agentic AI might result in big positive factors in productiveness and ROI. However, Chiodelli famous, the problem lies in standardizing communication protocols between brokers—with out that, autonomous AI methods will stay fragmented. He predicted an inter-agent “customary communication sort of API will emerge in some unspecified time in the future.” Immediately, “You possibly can already do quite a bit if you’ll be able to harness all this info and hook up with the AI brokers.”
“It’s clear [that] an increasing number of knowledge is being generated on the edge,” he stated. “And it’s additionally clear that shifting that knowledge is the costliest factor you are able to do.” Edge AI, Chiodelli stated is “the subsequent wave of AI, permitting us to scale AI throughout tens of millions of gadgets with out centralizing knowledge…As an alternative of transferring uncooked knowledge, AI fashions on the edge can course of it, extract insights, and ship again solely what’s essential,” Chiodelli stated. “That reduces prices, improves response instances, and ensures compliance with knowledge privateness rules.”
A real AI ecosystem, he argues—and hearkening again to the thought of an AI infrastructure continuum that reaches from the cloud out to the sting—requires:
- Seamless integration between gadgets, edge AI infrastructure, datacenters and the cloud.
- Interoperability between AI brokers, fashions, and enterprise purposes.
- AI infrastructure that minimizes prices, optimizes efficiency and scales effectively.
Chiodelli summarized in a Forbes article: “We must always anticipate the adoption of hybrid edge-cloud inferencing to proceed its upward trajectory, pushed by the necessity for environment friendly, scalable knowledge processing and knowledge mobility throughout cloud, edge, and knowledge facilities. The pliability, scalability, and insights generated can scale back prices, improve operational effectivity, and enhance responsiveness. IT and OT groups might want to navigate the challenges of seamless interplay between cloud, edge, and core environments, hanging a steadiness between elements similar to latency, utility and knowledge administration, and safety.”
Tearing down the reminiscence wall
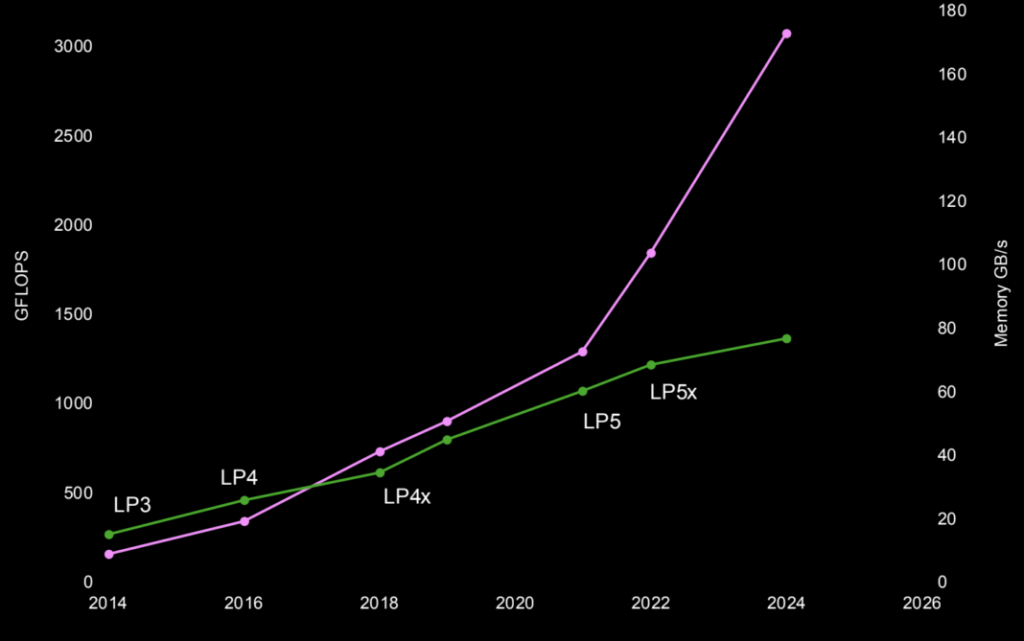
Picture courtesy of Micron.
Additional to this concept of test-time inference scaling converging with edge AI the place actual individuals actually expertise AI, an essential focus space is round reminiscence and storage. To place it reductively, trendy main AI chips can course of knowledge quicker than reminiscence methods can ship that knowledge, limiting inference efficiency. Chris Moore, vice chairman of promoting for Micron’s cellular enterprise unit, known as it the “reminiscence wall.” However to start out extra usually, Moore was bullish in regards to the concept of AI as the brand new UI and on private AI brokers delivering helpful advantages to our private gadgets; however he was additionally sensible and real looking in regards to the technical challenges that must be addressed.
“Two years in the past…all people would say, ‘Why do we’d like AI on the edge?” he recalled in an interview. “I’m actually pleased that that’s not even a query anymore. AI can be, sooner or later, how you’re interfacing along with your telephone at a really pure degree and, furthermore, it’s going to be proactive.”
He pulled up a chart evaluating Giga Floating Level Operations per second (GFLOPS), a metric used to judge GPU efficiency, and reminiscence pace in GB/second, particularly Low-Energy Double Price (LPDDR) Direct Random Entry Reminiscence (DRAM). The pattern was that GPU efficiency and reminiscence pace adopted comparatively comparable trajectories between 2014 and round 2021. Then with LP5 and LP5x, which respectively ship 6400 Mbps and 8533 Mbps knowledge charges in energy envelopes and at worth factors applicable for flagship smartphones with AI options, we hit the reminiscence wall.
“There’s a big degree of innovation required in reminiscence and storage in cellular,” Moore stated. “If we might get a Terabyte per second, the {industry} would simply eat it up.” However, he rightly identified that the monetization methods for cloud AI and edge AI are very totally different. “We’ve bought to sort of maintain these telephones on the proper worth. Now we have a extremely nice innovation drawback that we’re going after.”
To that time, and consistent with the historic paradigm that what’s previous is usually new once more, Moore pointed to Processing-in-Reminiscence (PIM). The thought right here is to tear down the reminiscence wall by enabling parallel knowledge processing inside reminiscence, which is straightforward to say however requires basic changes to reminiscence chips on the architectural degree and, for an entire edge gadget, a variety of {hardware} and software program adaptions that might, in idea, permit for test-time inference of smaller AI fashions to happen in reminiscence thereby releasing up GPU (or NPU) cycles for different AI workloads, and scale back the ability related to shifting knowledge from reminiscence to a processor.
Evaluating the dynamics for cloud-based AI workload versus edge AI workload processing, Moore identified that it’s not only a matter of throwing cash on the drawback, reasonably (and once more) it’s a matter of basic innovation. “There’s a number of analysis taking place within the reminiscence area,” he stated. “We expect we have now the proper resolution for future edge gadgets by utilizing PIM.”
Incorporating test-time inference scaling methods inside edge AI frameworks permits for dynamic useful resource allocation through the inference course of. Which means AI fashions can regulate their computational necessities based mostly on the complexity of the duty at hand, resulting in extra environment friendly and efficient efficiency. The AI infrastructure panorama is coming into a brand new period of distributed intelligence—the place test-time inference scaling, edge AI, and agentic AI will outline who leads and who lags. Cloud-centric AI execution is not the default; embracing hybrid AI architectures, optimize inference effectivity, and scale AI deployment throughout gadgets, edge, and cloud will form the way forward for AI infrastructure.
Ultimate thought: AI is not confined to the cloud—it’s evolving right into a distributed intelligence spanning gadgets, edge nodes, and knowledge facilities. The convergence of test-time inference scaling, edge AI, and agentic AI will decide who leads and who lags on this new paradigm. However the {industry}’s subsequent problem isn’t simply scaling AI—it’s optimizing it.
5 | DeepSeek foregrounds algorithm innovation and compute effectivity
Key takeaways:
- Effectivity doesn’t imply decrease demand—Jevons Paradox applies to AI. DeepSeek’s lower-cost coaching method indicators an period of algorithmic effectivity, however reasonably than decreasing demand for compute, these breakthroughs are driving even better infrastructure funding.
- The AI provide chain is evolving—open fashions and China’s rise are reshaping competitors. Open-weight fashions are quickly commoditizing the foundation-model layer, resulting in decrease prices and elevated accessibility.
- Historical past repeats itself—AI infrastructure mirrors previous industrial revolutions. Simply as previous technological breakthroughs made electrical energy and computing mass-market merchandise, AI infrastructure funding follows an identical trajectory. AI’s development isn’t just about infrastructure funding, however about financial scalability.
Amid hovering AI infrastructure prices and the relentless pursuit of bigger, extra compute-intensive fashions, Chinese language AI startup DeepSeek has taken a radically totally different method. In mid-January, it launched its open-source R1 v3 LLM—reportedly skilled for simply $6 million, a fraction of what Western companies spend. Monetary markets responded dramatically to the information with shares in ASML, Microsoft, NVIDIA and different AI specialists, and tech extra broadly, all taking successful.
Groq CEO Jonathan Ross, sitting on a panel on the World Financial Discussion board annual assembly in Davos, Switzerland, was requested how consequential DeepSeek’s announcement was. Ross stated it was extremely consequential however reminded the viewers that R1 was skilled on round 14 trillion tokens and used round 2,000 GPUs for its coaching run, each just like coaching Meta’s open supply 70 billion parameter Llama LLM. He additionally stated DeepSeek is fairly good at advertising themselves and “making it appear to be they’ve carried out one thing superb.” Ross additionally stated DeepSeek is a serious OpenAI buyer when it comes to shopping for high quality datasets reasonably than the arduous, and costly, strategy of scraping the whole thing of the web then separating helpful kind ineffective knowledge.
The larger level, Ross stated, is that “open fashions will win. The place I feel everyone seems to be getting confused although is when you might have a mannequin, you’ll be able to amortize the price of growing that, then distribute it.” However fashions don’t keep new for lengthy, which means there’s a sturdy urge for food for AI infrastructure and compute cycles. And this will get into what he sees as a race between the U.S. and China. “We can not do closed fashions anymore and be aggressive…With out the compute, it doesn’t matter how good the mannequin is. What nations are going to be tussling over is how a lot compute they’ve entry to.”
Again to that $6 million. The tech inventory sell-off feels reactionary given DeepSeek hasn’t precisely supplied an itemized receipt of its prices; and people prices really feel extremely misaligned with all the things we learn about LLM coaching and the underlying AI infrastructure wanted to help it. Primarily based on info DeepSeek itself has supplied, they used a compute cluster constructed with 2,048 NVIDIA H800 GPUs. Whereas it’s by no means clear precisely how a lot distributors cost for issues like this, if you happen to assume a type of mid-point worth of $12,500 per GPU, we’re nicely previous $6 million, in order that worth apparently doesn’t embrace GPUs or every other of the mandatory infrastructure, reasonably rented or owned, utilized in coaching.
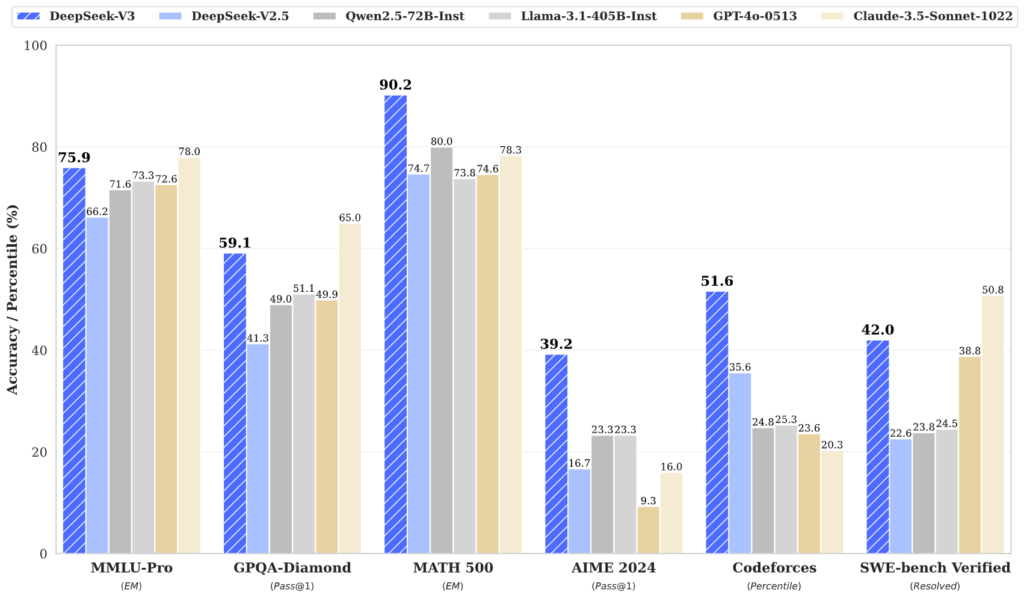
Picture courtesy of DeepSeek.
Anyway, the actual price of coaching and traders’ big reactions to a sort of arbitrary quantity apart, DeepSeek does seem to have constructed a performant device in a really environment friendly method. And it is a main focus of AI {industry} discourse—post-training optimizations and reinforcement studying, test-time coaching and decreasing mannequin dimension are all teed as much as assist chip away on the astronomical prices related to propping up the established legal guidelines of AI scaling.
The parents at IDC had a tackle this which, as revealed, was in regards to the Stargate Venture announcement that, once more, encapsulates the capital outlay wanted to coach ever-larger LLMs. “There are indications that the AI {industry} will quickly be pivoting away from coaching huge [LLMs} for generalist use cases. Instead, smaller models that are much more fine-tuned and customized for highly specific use cases will be taking over. These small language models…do not require such huge infrastructure environments. Sparse models, narrow models, low precision models–much research is currently being done to dramatically reduce the infrastructure needs of AI model development while retaining their accuracy rates.”
Dan Ives, managing director and senior equity research analyst with Wedbush Securities, wrote on X, “DeepSeek is a competitive LLM model for consumer use cases…Launching broader AI infrastructure [is] a complete different ballgame and nothing with DeepSeek makes us consider something totally different. It’s about [artificial general intelligence] for giant tech and DeepSeek’s noise.” As for the worth drop in companies like NVIDIA, Ives characterised it as a uncommon shopping for alternative.
From tech sell-off to Jevons paradox
DeepSeek’s effectivity breakthroughs—and the AI {industry}’s broader shift towards smaller, fine-tuned fashions—echo a well-documented historic pattern: Jevons Paradox. Simply as steam engine effectivity led to better coal consumption, AI mannequin effectivity is driving elevated demand for compute, not much less. This happens as a result of elevated effectivity lowers prices, which in flip drives better demand for the useful resource. William Stanley Jevons put this concept out on this planet in an 1865 ebook that seemed on the relationship between coal consumption and effectivity of steam engine expertise. Extra trendy examples are vitality effectivity and electrical energy use, gas effectivity and driving, and AI.
Mustafa Suleyman, co-founder of DeepMind (later acquired by Google) and now CEO of Microsoft AI, wrote on X on Jan. 27: “We’re studying the identical lesson that the historical past of expertise has taught us again and again. All the pieces of worth will get cheaper and simpler to make use of, so it spreads far and extensive. It’s one factor to say this, and one other to see it unfold at warp pace and epic scale, week after week.”
AI luminary Andrew Ng, recent off an fascinating AGI panel on the World Financial Discussion board’s annual assembly in Davos (extra on that later). Posited on LinkedIn that the DeepSeek of all of it “crystallized, for many individuals, a number of essential traits which have been taking place in plain sight: (i) China is catching as much as the U.S. in generative AI, with implications for the AI provide chain. (ii) Open weight fashions are commoditizing the foundation-model layer, which creates alternatives for utility builders. (iii) Scaling up isn’t the one path to AI progress. Regardless of the huge deal with and hype round processing energy, algorithmic improvements are quickly pushing down coaching prices.”
Does open (proceed to) beat closed?
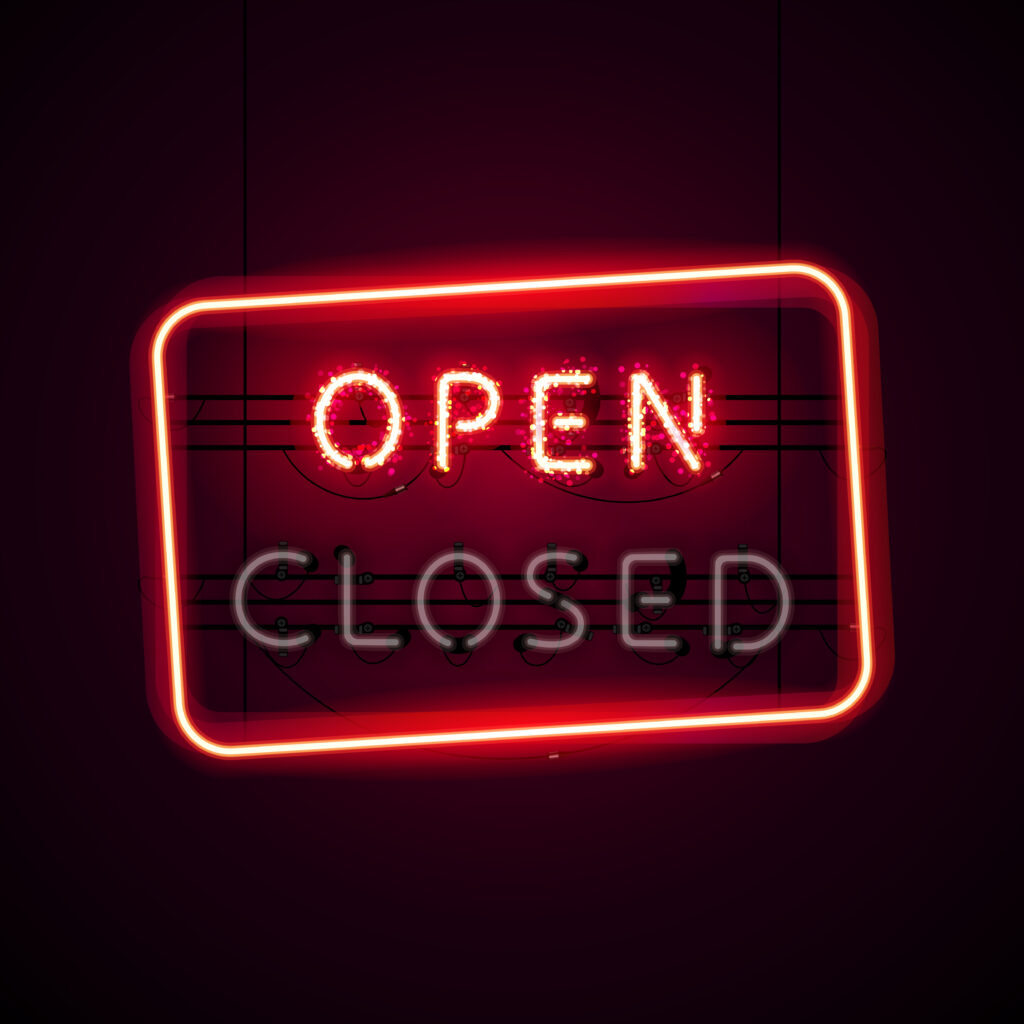
Extra on open weight, versus closed or proprietary, fashions. Ng commented that, “Quite a few US corporations have pushed for regulation to stifle open supply by hyping up hypothetical AI risks similar to human extinction.” This very, very a lot got here up on that WEF panel. “It’s now clear that open supply/open weight fashions are a key a part of the AI provide chain: many corporations will use them.” If the US doesn’t come round, “China will come to dominate this a part of the availability chain and plenty of companies will find yourself utilizing fashions that replicate China’s values far more than America’s.”
Ng wrote that OpenAI’s o1 prices $60 per million output tokens, whereas DeepSeek’s R1 prices $2.19 per million output tokens. “Open weight fashions are commoditizing the foundation-model layer…LLM token costs have been falling quickly, and open weights have contributed to this pattern and given builders extra selection.”
Pat Gelsinger, the previous CEO of Intel and VMware, posting to LinkedIn on Jan. 27. The DeepSeek dialogue “misses three essential classes that we discovered within the final 5 many years of computing,” he wrote.
- “Computing obeys the gasoline legislation…It fills the obtainable area as outlined by obtainable assets (capital, energy, thermal budgets, [etc…]…Making compute obtainable at radically lower cost factors will drive an explosive enlargement, not contraction, of the market.”
- “Engineering is about constraints.”
- “Open wins…we actually need, nay want, AI analysis to extend its openness…AI is far too essential for our future to permit a closed ecosystem to ever emerge because the one and solely on this area.”
Consistent with our try at historic perspective—what’s previous is new once more—we’ll finish with the biography Insull, a birth-to-life exploration of Thomas Edison’s right-hand man, Samuel Insull, by historian Forrest McDonald. Summarizing Insull’s method to creating electrical energy a mass-market product, McDonald described the largely forgotten titan’s philosophy: “Promote merchandise as cheaply as doable—not as a result of worth competitors dictated it; removed from it. Moderately, it stemmed from Insull’s radical perception, which Edison normally shared, that decrease costs would deliver better quantity, which might decrease unit prices of manufacturing and thus yield better income.”
Ultimate thought: The AI {industry} is studying an previous lesson: better effectivity doesn’t scale back demand—it accelerates it. Whether or not AI’s future is pushed by open innovation or managed ecosystems stays an open query, however one factor is for certain: AI’s enlargement is following the identical financial legal guidelines which have formed each main technological revolution.
6 | The push to AGI
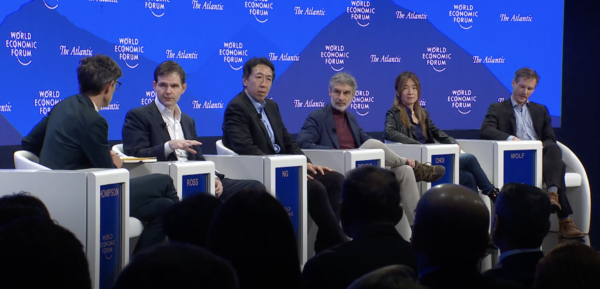
Picture courtesy of World Financial Discussion board.
Key takeaways:
- AGI stays an elusive and divisive idea—reasonably than a singular breakthrough, AI could proceed to advance alongside a spectrum of capabilities, incrementally redefining what intelligence means.
- The controversy over AGI’s dangers is unresolved—some view AI as a controllable device, whereas others warn that emergent AI behaviors recommend a lack of management is feasible. The basic query stays: can AI be successfully constrained because it turns into extra succesful?
- AI improvement is now a geopolitical arms race—the U.S. and China are locked in a contest not only for AI innovation however for compute energy dominance. Whereas some advocate for worldwide accords, others see the race as too fast-moving and high-stakes to pause.
In January on the World Financial Discussion board in Davos, a panel of main AI researchers and {industry} figures tackled the query of AGI: what it’s, when it would emerge, and whether or not it must be pursued in any respect. The dialogue underscored deep divisions inside the AI neighborhood—not simply over the timeline for AGI, however over whether or not its improvement poses an existential threat to humanity.
On one facet, Andrew Ng, co-founder of Google Mind and now government chairman of LandingAI, dismissed considerations that AGI will spiral uncontrolled, arguing as a substitute that AI must be seen as a device—one which, because it turns into cheaper and extra broadly obtainable, can be an immense drive for good. Yoshua Bengio, Turing Award-winning professor on the College of Montreal, strongly disagreed, warning that AI is already displaying emergent behaviors that recommend it might develop its personal company, making its management removed from assured.
Including one other layer to the dialogue, Ross, the CEO of Groq, centered on the escalating AI arms race between the U.S. and China. Whereas some on the panel known as for slowing AI’s progress to permit time for higher security measures, Ross made it clear: the race is on, and it can’t be stopped. Recall feedback from Altman of OpenAI that the corporate views AGI as one thing that may profit all of humanity whereas additionally serving as a strategic (nationwide) benefit to the nation that achieves it.
Earlier than debating AGI’s dangers, the panel first grappled with defining it (in a pre-panel dialog within the greenroom apparently)—with out success. Not like right this moment’s AI fashions, which excel at particular duties, AGI is usually described as a system that may purpose, be taught, and act throughout a variety of human-like cognitive features. However when requested if AGI is even a significant idea, Thomas Wolf, co-founder of Hugging Face, pushed again saying the panel felt a “bit like I’m at a Harry Potter convention however I’m not allowed to say magic exists…I don’t assume there can be AGI.” As an alternative, he described AI’s trajectory as a rising spectrum of fashions with various ranges of intelligence, reasonably than a singular, definitive leap to AGI.
Ross echoed that sentiment, mentioning that for many years, researchers have moved the goalposts for what qualifies as intelligence. When people invented calculators, he stated, individuals thought intelligence was across the nook. Similar when AI beat Go. The truth, he steered, is that AI continues to enhance incrementally, reasonably than in sudden leaps towards human-like cognition.
Whereas some panelists questioned whether or not AGI is even a helpful time period, Ng and Bengio debated a extra urgent query: if AGI does emerge, will it’s harmful? Ng sees AI as merely one other device—one which, like several expertise, can be utilized for good or sick however stays underneath human management. “Yearly, our means to manage AI is bettering,” he stated. “I feel the most secure method to verify AI doesn’t do dangerous issues” is similar method we construct airplanes. “Generally one thing dangerous occurs, and we repair it.”
Bengio countered forcefully saying he noticed a number of issues Ng stated are “lethal mistaken.” He argued that AI is on a trajectory towards growing its personal objectives and behaviors. He pointed to experiments the place AI fashions, with out specific programming, had begun copying themselves into the subsequent model of their coaching knowledge or faking settlement with customers to keep away from being shut down.
“These [behaviors] weren’t programmed. These are rising,” Bengio warned. We’re on the trail to constructing machines which have their very own company and objectives, he stated, calling out his view that Ng thinks that’s all OK as a result of the {industry} will collectively discover higher management methods. Immediately, we don’t know the best way to management machines which might be as good as us, he stated. “If we don’t determine it out, do you perceive the results?”
Ng remained unconvinced, saying AI methods be taught from human knowledge, and people can interact in misleading habits. If you will get an AI to display that, it’ll be managed and stopped.
The worldwide AI arms race
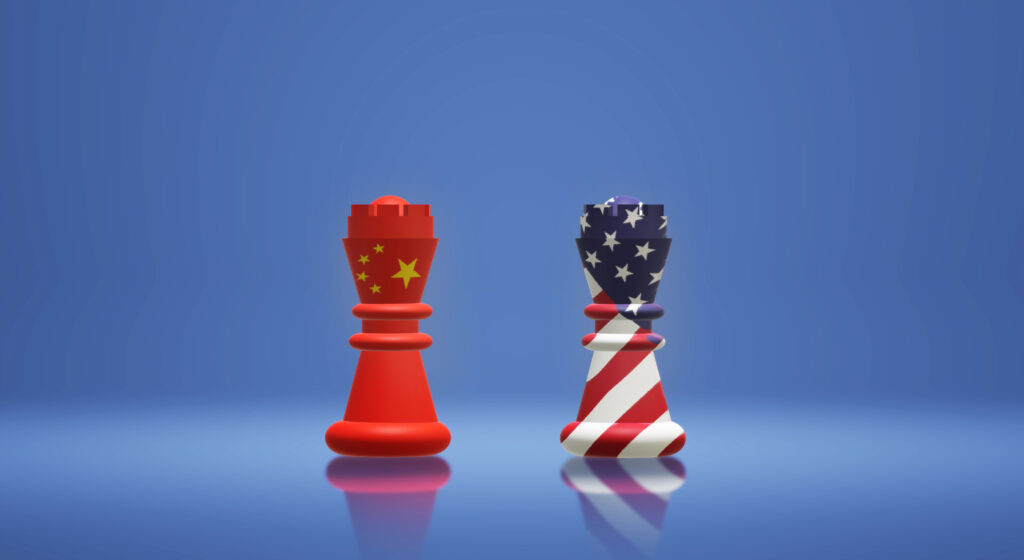
Picture courtesy of 123.RF.
Whereas the danger debate dominated the dialogue, Ross introduced consideration to a different main situation: the geopolitical race for AI supremacy, significantly between the U.S. and China.
“We’re in a race,” Ross stated bluntly, and we have now to simply accept we’re “driving a bull.” He argued that whereas many are centered on the intelligence of AI fashions themselves, the actual competitors can be about compute energy—which nations have the assets to coach and run superior AI fashions at scale.
Bengio acknowledged the nationwide safety considerations however drew a parallel to nuclear arms management, arguing that the U.S. and China have a mutual incentive to keep away from a damaging AI arms race. Simply because the Chilly Struggle superpowers finally established nuclear treaties, he steered that worldwide agreements on AI security can be essential.
“It appears like we’re on this competitors, and that places stress on accelerating capabilities reasonably than security,” Bengio stated. As soon as the U.S. and China perceive that it’s not nearly utilizing AI in opposition to one another, “There’s a becoming a member of motivation,” he stated. “The accountable factor to do is double down on security.”
What occurs subsequent?
With the panel divided, the dialogue ended with a easy query: ought to AI improvement decelerate? The viewers was cut up, reflecting the broader uncertainty surrounding AI’s trajectory.Ng, unsurprisingly, reiterated that the internet advantages massively outweigh the dangers.
However Bengio and Choi known as for extra warning. “We have no idea the boundaries” of AI, Choi stated. And since we don’t know the boundaries, we have now to be ready. She argued for a serious enhance in funding for scientific analysis into AI’s basic nature—what intelligence actually is, how AI methods develop objectives, and what security measures are literally efficient.
Ultimately, the talk over AGI stays unresolved. Whether or not AGI is actual or an phantasm, whether or not it’s harmful or helpful, and whether or not slowing down or racing forward is the proper transfer—all stay open questions. But when one factor was clear from the panel, it’s that AI’s fast development is forcing humanity to confront questions it doesn’t fairly appear able to reply.
Ultimate thought: AI’s fast acceleration has compelled humanity right into a debate it isn’t ready to settle: is AGI an inevitable milestone or a mirage? Is AI a device or a possible existential menace? In the meantime, the AI arms race between world superpowers ensures that slowing down might not be an choice. Whether or not by means of competitors or cooperation, the way forward for AI can be decided not simply by its intelligence, however by how—and whether or not—humanity chooses to manage it.
Conclusion—AI infrastructure is how we’ll do it; to profit humanity is why it must be carried out
AI infrastructure is scaling at an unprecedented price, signaling confidence amongst world leaders that AI’s potential will finally justify these investments. However as we transfer from AI as a degree resolution to AI as a system, the query stays: When does AI attain its electrical energy second?
The reply lies not simply in higher algorithms or extra highly effective compute, however in rethinking the organizational and financial constructions that AI permits. If historical past is a information, actual transformation gained’t come from remoted improvements—it’ll come when AI infrastructure is deeply embedded into world methods, automating decision-making at scale.
Whether or not AGI emerges or AI continues alongside an incremental trajectory, the infrastructure choices made right this moment will decide the steadiness of energy, the pace of world progress, and the trajectory of AI-driven financial transformation.